The Pressing Demand for Generative AI in Enterprise
Generative AI (GenAI) is promising unparalleled advancements and efficiencies for many types of use cases. Boards and CEOs continue to experiment with the technology and imagine how it can improve workforces and increase throughput. The Wall Street Journal highlights how CEOs are pressuring CIOs and technology leaders to urgently install generative AI for fear of being left behind and CIOs are feeling the heat. However, with the dynamics and complexity of adopting generative AI in enterprise settings, it becomes clear that managing expectations is just as important as it is about technological integration.
Generative AI Sets False Expectations
The simplicity and efficiency of generative AI in personal use often paint an unintentionally misleading picture in an enterprise setting. When CEOs and other non-technical leaders personally interact with tools like ChatGPT, they’re introduced to the potential of the technology in an uncomplicated, straightforward context. This magical experience often sets false expectations, leading them to question why such technology isn’t already integrated into the broader systems of their companies. However, the reality is that scaling these tools for enterprise needs is a vastly more intricate process. It’s akin to the difference between cooking a meal for oneself versus catering for a large event with complex dietary restrictions; the underlying task is the same, but the scope and complexity are dramatically different. This lack of understanding between personal uses and the intricacies of enterprise deployment highlights the need for clearer communication about the capabilities and limitations of AI tools in a business context.
The Intricacies of Enterprise Implementation
Deploying generative AI in an enterprise setting is more than meets the eye. While individuals might find generative AI to be a convenient solution for isolated tasks, integrating it within a business’s broader systems demands addressing a series of complex challenges. As John points out while a user might see generative AI as solving 100% of a personal problem, it only covers about 5% of the challenges in a business context. The vast majority of the work comes from:
- Content ingestion: Importing data correctly is a massive challenge, especially when dealing with varied content like text, tables, images, and metadata. Properly importing, categorizing, and managing this data is a colossal task that requires precision to ensure you prompt an AI model with the right context and information.
- Real-time access: Unlike personal use scenarios, where static data is sufficient, enterprises operate in dynamic environments and require real-time data, which means integrating AI models with existing systems in a nimble and adaptable method.
- Data security: Enterprises deal with vast amounts of sensitive data, and any AI model must operate securely within existing frameworks, ensuring that access is limited to only the appropriate roles and parties.
- Scalability and cost: Experimenting with public interfaces is free or inexpensive but deploying these models at scale can be extremely costly so enterprises need to be able to manage these costs and justify the investments.
The Perilous Path of Doing It Yourself
There’s often a pioneering spirit among IT departments—a drive to create, to innovate, and to build from the ground up. This behavior, while commendable, can be a treacherous path when it comes to AI solutions like generative AI. The challenge with integrating a single model or trying to build or train your own lies not just in the initial creation and training, but in the ongoing maintenance, updates, and inevitable troubleshooting. Unlike traditional software applications, AI models are dynamic and require continuous feeding of data, iterative refining, and adjustments based on evolving algorithms. Therefore, the conventional approach of building one’s applications, which many IT organizations are accustomed to, is not suitable for AI implementations. This method is not only time-consuming but increases the risk of accumulating technical debt. Each customization, tweak, or workaround can lead to future complications that slow down operations and make subsequent adjustments more difficult. It’s essential to recognize that while bespoke solutions might offer a veneer of control, the real power in AI lies in leveraging platforms that enable subject matter experts to modify workflows on the fly versus submitting feature requests to IT that become obsolete before the fix is deployed.
Adjusting Expectations for Generative AI
Generative AI advancements have elevated stakeholders’ perceived possibilities and achievable short-term desires. Now, CIOs find themselves managing executive expectations with the realities of securely integrating new AI capabilities. They must align the thrill generated by generative AI’s potential based on personal experiences, with the complex challenges of enterprise deployment. Non-technical stakeholders may appreciate AI tools’ simplicity and efficiency without fully understanding the intricacies of large-scale deployment with new technology that is still in its infancy. Successful integration means aligning the tool with existing systems, adhering to data security standards, and ensuring cost-effectiveness. Therefore, CIOs and tech leaders need to prioritize transparent communication to educate stakeholders about AI complexities and set realistic deployment timelines and have to do this faster than ever before.
The Road Ahead with Generative AI
Integrating AI in your enterprise requires more than just enthusiasm—it demands a strategic approach. The seemingly all-knowing and simple question-and-answer experiments on public interfaces must be paired with understanding the complexities of scaling them for larger, organizational needs. It’s not merely about embracing the technology; it’s about integrating it in a manner that governs context, data security, cost implications, and adaptability as AI advances. Integrating generative AI into your business isn’t just a choice—it’s a commitment.
To successfully integrate generative AI into your enterprise, keep these steps in mind:
- Start by identifying a couple of use cases where the benefits outweigh the risks and have a clear understanding of them. Don’t rush to deploy generative AI everywhere, but rather focus on high-impact areas with a strong champion.
- Launch controlled projects based on these use cases. This way, you can demonstrate the capabilities and value of generative AI, quantify the outcomes, and build momentum for secondary use cases.
- Establish strong data governance protocols taking into account Intellectual Property protection and role-based access.
- Provide ample training to your workforce. Empower your employees to effectively interface with AI tools enabling better human-AI collaboration and building trust.
- Always monitor the performance of the AI models and user feedback after deployment. This way, you can identify any issues and modify or interchange models as advancements occur.
The journey towards integrating generative AI in your enterprise is simple if you plan effectively and leverage the right tools. It involves more than simple adoption—it demands understanding, strategic planning, careful deployment, and continuous assessment. With the right approach, clear use cases, strong data governance, skillful training, and vigilant monitoring, generative AI can be effectively integrated to drive considerable value to your business, fostering innovation, and giving your organization a competitive edge.
Links and Resources
- CIOs Feel Heat From CEOs on Generative AI, The Wall Street Journal
- The organization of the future: Enabled by gen AI, driven by people, McKinsey & Co.
- How to Choose an LLM, Krista Software
Speakers
Transcript
Scott King:
Well, hey everyone, thanks for joining. I’m Scott King and that is John Michelson. Hey John. John’s recently our Chief Product Officer after running Krista Software for some years. And we’re always glad to have you John because most of our ideas and most of our content come from what you think is gonna happen four or five years from now. So really appreciate you being on the podcast today. We’re going to talk about the juxtaposition between how we personally use the gen AI products, ChatGPT, Bard, and what have you, versus what happens when you bring it inside, right? CEOs are pressuring the technology leaders to, hey, I used ChatGPT over the weekend. It’s really cool. Why aren’t we already doing this, right? There’s a great Wall Street Journal article that came out a couple of days ago, the CIO’s Feel the Heat. But what you don’t understand is kind of their excuse. There are lots of articles. McKinsey talks about culture change and the transformation of people and things like that. But to begin with, you’ve been CEO a couple of times, and you’ve been on boards of directors, What’s the conversation at the board level with the CEO and then shoving this down to the tech leaders like the CIO? Like, why aren’t you done yet? This thing’s so easy.
John Michelsen:
Yeah, well, so when you use a chat GPT or any of the models personally, you just jump on a website and you say, hey, I want to decline the party to Scott. He was going to, it was a costume party and blah, blah. And it generates something and you’re going to edit that response just a little bit, copy paste it into an email and go. And then you’re going to think, wait…I just used gen AI to respond to emails. Why don’t I go ask my contact center leader to just get gen AI to respond to all our inbound emails when we’re done? And why isn’t that? I mean, I just did it. It was so easy, right? I mean, can we see it at noon? Right? There’s a certain understandable euphoria around new tech and we can always, you know give proper credit to Gartner for the hype cycle of, I think, best describing that whole idea. And we’re at the tip of the top of that, I think. Yeah. Well, I’m glad you’re here.
Scott King:
Yeah, I think we’re at the peak of whatever it is. Yeah, I think we’re about there.
John Michelsen:
Yes, indeed. The goal is one we all share, right? It’s not as if the contact center leader, the CIO, the supply chain leader, or the sales leader doesn’t yearn for the same experience. An experience that is so easy and speedy that it brings immediate value. And why not? There’s a straightforward answer to this. The answer is not to avoid the challenges that I’m about to bring up. The fact is, there’s an immeasurable value to be unlocked. However, there’s an approach that will work, and then there are many that will require a lot of effort and will attempt to morph your organization into something it is not. Hopefully, we can cover all of that within the timeframe of this podcast.
I would summarize the difference, that juxtaposition as you’ve described it, as such: you asked the LLM to draft an email to decline an invitation. You saw it as a complete solution to your problem. You simply copied and pasted it on your own, et voilà! You thought, “Gen.ai has provided 100% of the solution I needed to perform this task.” However, in reality, in an enterprise context, when you’re providing a question-answer type of capability to an audience, it constitutes less than 5% of the total effort that is required. Now, why is that? Why is it that it’s 100% of the personal solution, but it’s only 5% of the enterprise solution?
Scott King:
You mean the gen AI piece is only 5%? Yeah, OK.
John Michelsen:
That’s right. But the truth lies entirely in your declining of the invite. How so, you may ask? Well, it’s quite straightforward. When you used it personally, you already established the context—it was in your head. You told it precisely what you were looking for. You provided it with the answer. Not “should I go?” or “should I not go?”, but “I need to decline.” You gave it the context it needed, presumably in order to properly decline. A human, in this case you, read the response, found it to be clever, likely made some changes, and then put it into an email. So there was still a human in the loop, although you might not have thought of it that way. However, that’s exactly what happened. You might perceive it as 100% of the solution. In reality, even in your personal use, it’s not 100% end-to-end. In an enterprise context, it’s far less—it’s more like 5%. And the differences are vast.
Let’s try to skim the surface of these differences. Then, perhaps we can delve deeper into one or two of them, depending on what the audience might prefer. Scott, what do you think?
Scott King:
All right, so you’re describing the remaining 95% to us. OK.
John Michelsen:
Absolutely. We have already helped customers create and fill out several RFPs in this very type of motion. The actual LLM questions are among the 30, right? The LLM itself is really about the evaluation of the appropriate Large Language Model. The gen AI technology and GEN.AI as a field is actually not just comprised of large language models, or foundation models. It consists of many other AI capabilities, algorithms, and content, and even includes traditional data processing tasks that need to be solved.
But let’s delve into that. First, as an individual, you tell the model,” This is what I need you to do”. The context comes from a human who sent you an email or the inbound request from one of your reps to understand the compatibility of two different products in your catalog, among other things. That context has to be constructed, so you ingest content into a system, not directly into an LLM, but into a system. The ingestion of content continually reminds us of the challenges of ‘garbage in, garbage out’ and the quality of the content plus the quality of the ingestion of that content. Tables, images, graphs, and obviously text need proper structure, tone, and metadata around each file. The relative appropriateness of that content versus other content challenges us even getting to the right answer.
You gave the LLM the answer. You said, “I’m declining.” You didn’t ask the LLM whether to decline or not. You have to tell the LLM the answer. We don’t think of it that way. We think it already knows the answers. Because we personally sort of gave it the answer – the answer is I’m not going. What I need you to do is just construct a nice, elegant way for me to get out of the party. That’s easy. I’m not demoting the value of an LLM. It’s cool stuff. But it first needs to be given an answer.
A perfect example – you’re the sales manager, and you need to know the current pipeline in your region that has a likelihood to close this quarter so that you can focus on it, right? That’s not cached Google results from some previous time. That’s going to be a live invocation of a system. You’re going to be doing an enormous amount of content ingestion long before you get to an LLM. That is very sophisticated work in its own right. You would think if you had it all sitting in PDFs or Word documents or something similar, it should be easy. But it’s actually a significant amount of work to make sure you’ve got that right. And it’s going to expose all the holes in your content, where humans currently fill the gaps.
So, there’s a lot related to content ingestion. There’s a lot related to real-time access to information because most of what people ask aren’t just a surrogate for a search of existing static content. The questions that make Gen.i powerful aren’t just as easily answered with an enterprise Google-type search, but to actually go and integrate real-time information that people need and marry that with that static information.
So, I need to know the part, the suppliers we use for product XYZ’s companion parts. You just possibly went into one or two systems because maybe you referenced a particular proposal, the parts on a particular proposal. You accessed real-time information through a CRM or sales order management or what have you. Maybe you even went into the supply chain system to get a bill of materials for that particular product, and now you’re into static content that is actually the reference material of those two intersections in order to get that. So I’m going super fast, and I apologize if I’m losing people in the process, but what I’m trying to help you recognize here is, yeah, you can give it the answer, and tell it to give me a clever way to provide a response.
But the clever answer part in the enterprise, not as easy as I wanted to, I’m just telling you I’m declining. It’s actually a significant effort in its own right. And to get the content right, especially when that content becomes significant, you can prompt an LLM with a certain amount of content, but not the entire body of content you have. You have to be very thoughtful about what content goes in. It’s not actually possible to go truly end-to-end, even in personal use – it’s not 100%. In the enterprise context, it’s even less, perhaps around 5%. The differences are vast.
I will try to skim across the top of these and then maybe drill down on one or two of them, depending on what you think the audience would prefer to learn more about, Scott.
Scott King:
All right, so you’re going to describe the remaining 95% to us. OK.
John Michelsen:
Exactly. Long before you even get started, we’ve already helped customers create and fill out several RFPs in this very type of motion. The LLM-related questions are just one of the 30. The LLM itself is really about the evaluation of the appropriate LLM in a large language model. The GenAI technology and GenAI as a space is not just large language models or foundation models, but also includes lots of other AI capabilities and algorithms, lots of other content, and even old-fashioned data processing stuff that you have to solve for.
First, as an individual, you tell the model what you need it to do. The human who sent you an email or the inbound request from one of your reps to understand the compatibility of two different products in your catalog, whatever it is. That context has to be constructed so you can ingest content into a system, not into an LLM by the way, but into a system. This ingestion of content continually reminds and what content does not go in. And that is not even just a, oh yeah, I heard about these embeddings things and you just do embeddings. Because frankly, embeddings are very, very poor when there are variations in content and type. Tables versus pros, images, small pieces of content versus large pieces of content. Your real-time information is gonna end up, typically, small bits of information, sitting beside very large chunks of information that are produced, let’s say, PDFs and Word documents, you got to make sure that all of that stuff gets properly analyzed correctly. Then there’s all kinds of AI and there’s all kinds of algorithm approaches to try to solve for that. But that is all outside the scope of the competency of most organizations. If you’re not a big AI shop doing a ton of NLP work like we’ve done for five, six years now.
It’s just not in the realm of, oh, we do this so well, and we’ve got all of this stuff. But what’s happening today a lot is, oh, I downloaded an open source framework that gets me started, and now I got a half a dozen developers plus working on it. This is where I think we’re going worse. This is where I think we’re going really, really poorly here because we’ve just decided we’re gonna build our own payroll system because we have to calculate taxes. Like, no, we do not, right? You guys, no one run in a business. It’s…
Scott King:
It does seem like people would go that direction because they have a big IT staff, and they’re used to developing their own software. It makes sense that they would try this, but the alternatives maybe are still unknown to them, right?
John Michelsen:
And well, it’s a wide open space. It is early. And you do need to get moving. So of course, the motivation is all there. And if you don’t have a good look at the market of, OK, are there ways I can change the approach? If I take the same old software development, let’s hand code a whole bunch of stuff and let’s solve every one of these problems that has already been solved before, yet again, instead of borrowing the capabilities and the learnings of others already.
You know, as simply as I could put it, I referenced the payroll system, because it’s a pretty obvious choice. It’s very hard to imagine someone wanting to go build a payroll system, and everybody needs to do payroll. You aren’t really in the best position if you’re trying to do all of this ground up. If you’re trying to build all of that 95% bespoke, you’re just signing yourself up for an enormous amount of challenges. And as I said, I referenced the RFPs that we’ve worked on recently. And it’s just a really good example. What about re-rankers? What are you doing about, you know, what type of embedding engines are you using? What vector databases are you using? And how are you doing X, Y, and Z? And what are your algorithms for this and that? And by the way, most of these RFPs are people just doing Google searches and have no idea what they’re even talking about, but they saw this Lang chain discussion on it, so they decided to put a question in, because as soon as I actually address it with them,
You realize they actually don’t understand what they’re actually even trying to go through. So the people that are trying to even build this stuff, using it all for the first time, not well-versed in any of these technologies and approaches, it just seems like a recipe for a disaster. And therefore, in time, I think we’ll find it’s just totally real, it’s totally rational to think.
I wouldn’t build my own platform for doing ERP. I wouldn’t build my own platform for doing payroll. I wouldn’t build my own platform, name your system. I wouldn’t build my own platform for doing generative AI. Don’t know why anybody would. Unless that’s your business, and if it is, then I hope you’re great at it. It is our business, we are great at it. Why not borrow the capability that’s already there? So I’ll try to pause it right there, because there’s just an enormous number of things that.
Unfortunately, you discover by realizing you’re delivering bad results. One real simple example, very good looking PDF file, completely poor results when you convert it in whatever way you convert it into content that then you ingest into that other 95%, right, the content management aspect of this, and then you prompt a model with it. And it’s giving you bad answers and you have no idea why and then you figure out the reason is because it’s very difficult for images, tables, titles, and etc. in a PDF to actually get correctly structured into that content repository. And that’s a heavy lift. And most of you guys are probably thinking, well, why don’t I just put everything in as a PDF? Well, actually that’s, unfortunately, not the best structure of content. Of course, we have to deal with it and we do the best we can. But that’s a long effort of discovery, of refinement and all of that stuff. Don’t start now and think, the pressure on you is, can we have something at noon and you’re going to be telling them about how it’s hard to ingest PDFs? That’s, you know, and why is this thing wrong? Well, let me tell you about how portable document format is actually really print document format and it’s actually not designed for, you’re going to totally lose your audience and you’re just going to say, why can’t I do this? I was able to decline a meeting.
So we’re fighting the, we’ve got 95% of the work to do before we ever get to the 5%. And your non-technical audience isn’t really gonna be that sophisticated at understanding.
Scott King:
So in that line of thought, the non-technical versus the technical, how do CIOs or VPs of tech, whoever is going to be running this, how do they reset the expectation of, I know you tried this, but, right, and there’s all these reasons, right? So what’s some of the, how would you respond in that type of situation?
John Michelsen:
Yeah, well, I guess if being a little self-serving. I suppose playback our podcast here at 0.5% instead of 1.5, like I normally do since I’m talking so fast at this particular moment. Use the analogy that we just gave, right? Yes, you can absolutely shove some content in, tweak it yourself, and get it to do something. That’s great that you can, but we in an enterprise have a whole different set of expectations and accuracy is paramount, of course.
It’s not the only one. There’s data security. There’s the performance of it, obviously, and the cost. Because it’s free for me to play around with it. But as soon as I put it in front of my entire customer base, you’re going to get a bill. Standing up an LLM is a very expensive proposition. Accessing one remotely is not as much at the moment in a few cases, because they’re eating a lot of cost and losing a ton of money. This is like, pardon me, but Uber all over again. Uber was a tenth the price of the taxi, and now it’s more than the taxi was a few years ago, right?
So we eventually have to support the LLMs that are currently getting their money, not through the front door of customers paying for it. And that will happen, right? So all of these things need to be, you can address them. I would focus more on we’re taking a superior approach. We’re going to get there faster than our competitors and we’re gonna move at a faster pace. Because unlike them, we’re not going with this ‘let’s build a big software development team, let’s go figure all of this out on our own, let’s go hiring a bunch of data engineers and let’s go figure out how hard this really is’ approach. Let’s go leapfrog all those guys and get somebody who’s already done this 30 times. Let’s be one of those who are getting the benefit of the continuous innovation of that at a really, really nice pace.
Of course, that’s with something like Krista, and we’d be happy to invite you to do that. Even if you were choosing to do this on your own, all I would say is to recognize that you’re starting a long journey here. You’ve got a very typical software development project with atypical results. LLMs tend to get fickle and not answer the same way, and your users say that your software’s wrong.
And there’s all kinds of things that you will have to be sorting through. So I don’t envy you the journey. I totally understand it. But I hope you’ll look to those who are already producing this solution that you can consume and of course customize it as you wish.”
Scott King:
Yeah, I appreciate it, John. The juxtaposition between doing it quickly in the hard way versus understanding all of those different requirements you’ve mentioned – data, performance, accuracy, and cost – is intriguing. The cost aspect may surprise people. For instance, the chat GPT, if you choose to pay for it, costs 20 bucks. But that’s for just one person.
John Michelsen:
Yes, that’s right, Scott. Here, we’re talking about a less-than-40-hour conversation where one of our partners is deploying this particular solution of ours into their entire customer base. And those customers are all coming back with the same response: their InfoSec teams are saying there’s no way they’re going to use a large, public language model.
Scott King:
Yes, a low number of tokens are going back and forth, so it’s inexpensive. But when you consider the grand scale of things, those costs could explode.
John Michelsen:
No, we’re not even considering using a shared server. You’ve got to set up one for us. Alone, Amazon’s charges are tens of thousands of dollars a month just to meet their performance and volume requirements. They’re requiring millions of these transactions, millions of business-level transactions, which might mean many lower-level transactions per business transaction. We’re talking about tens of thousands of dollars in Amazon EC2 charges each month. They just consider it as another EC2 box. Most data centers don’t even understand how to set up AI servers because it’s not just about power and heat challenges in regular servers. AI servers have a 10x power and heat problem due to how hot they run and how much power they draw.
The data center guys are not prepared to handle these kinds of things at all. Moreover, a typical large company probably has around 60 projects that all are setting up AI servers, trying to figure out how they’re going to proceed. So, there are lots of significant challenges associated with really getting generative AI right. It’s about taking a platform approach.
Coalescing onto a single stream of effort that is already more mature than you’re even thinking about, and riding that wave, seems to be the best way forward. However, I definitely notice a lot of people going about it the wrong way, or at least a much harder road to their destination.
Scott King:
The bumpier road, right? I think the road less traveled is going to be the simpler solution. Most people, I think, are going to go the wrong way.
John Michelsen:
They’ll start that way because that’s the course of action that is known, right? And it does make sense. There is a natural reaction to the need to deliver a new solution. I need to appoint a project manager, get an architect, and start building it. That architect’s natural inclination is that software costs money. Somehow, they think that they, and the next 12 people they’re about to hire, are free. I’m going to now deliver this solution, right? Compared to buying a product. Obviously, we can’t do that, but we did just open 10 or 12 RECs for people.
By the way, it’s not a zero-person thing, obviously, to deploy any kind of platform and solution. So there is an effort there, for sure. There are people to bring into your organization to really own this and get this right. But it’s an order of magnitude difference, literally an order of magnitude difference, like a 1 to 10 difference. We even have customer examples of that. I don’t know if we’ve mentioned on this blog that we were told by a customer they’re going from a 250 person hiring plan for next year to a 100 person hiring plan. The difference was what they saw. They already had problems on the drawing board, but they didn’t even know what they were going to do to solve. They saw them solved already, and they thought, ‘I can reset my hiring plan based on that.’
That’s a good thing, because frankly, they’re not going to get 250 people competently hired in this. There aren’t that many people. And of course, we’re going to see the job requisition that says, ‘Hey, you need five years of experience with OpenAI and Bedrock and blah,’ right? While they’re literally months mature enough to be used in a commercial context. I know this has gone long, but I hope our audience has appreciated at least the notion that I’m trying to help them see why there is this euphoria. It’s got to be so simple. I just used it. Why aren’t we doing it? And there is a mandate to get this thing delivering value in an organization. We’ve got great examples of customers who are doing it with phenomenal results.
All that said, just like most things in life, if you don’t consider thoughtfully how you might best get there, you might take the immediate, simple approach that you think or the approach you think is simple because it’s the one you already know. It might not be the right one, and I think if we’re more thoughtful, we will find that there’s a fantastic opportunity to leverage gene AI, along with, by the way, again, the 95% is dozens of other AI techniques. Integration of people and other systems are necessary to really pull this off. I think if you’re thinking that way, you might actually have something. It’s not going to be done by noon. It’s not like a CEO comes and sees you at 8 in the morning and you’re done at noon. You would actually have something done at noon, but there’s a lot that goes into this. Most of it’s even on the ‘what is the process? How would we and who would we give that information to?’ Those are good questions and those obviously do take time.
Scott King:
Alright, well thanks, John, I appreciate it. You know, greater detail obviously than we expected, but it’s always great talking with you and I really appreciate it. And until next time.
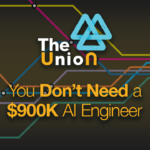
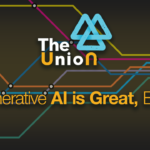
Read our comprehensive LLM testing guide
See how several LLMs fared in our test and steps to run your own tests for each of your use cases.
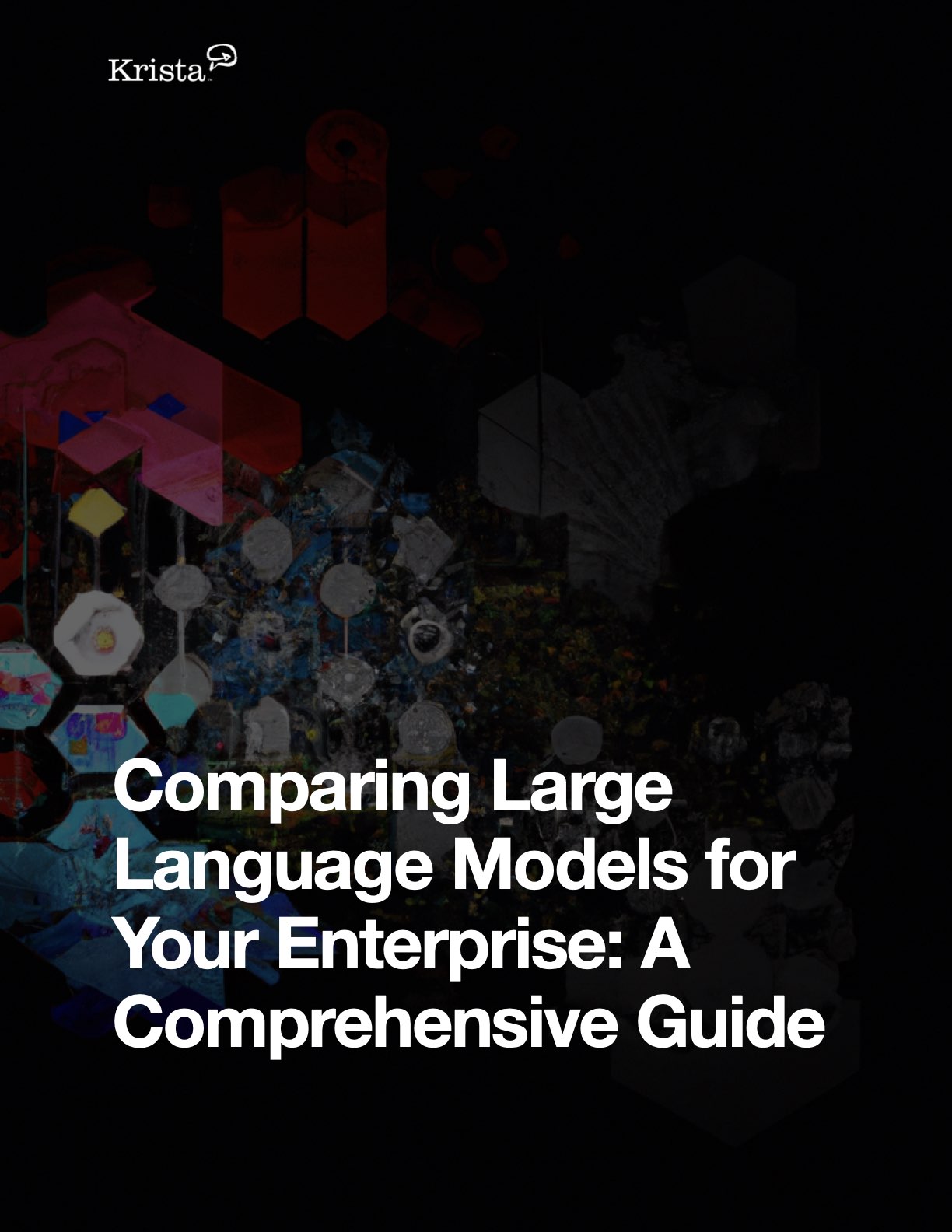