Generative AI vs Predictors and Categorizers
Generative AI is hot and has ignited our imaginations. However, it’s important to highlight that there are other AI capabilities, like predictors and categorizers, that can produce significantly more value, particularly in enterprise settings. But, these capabilities aren’t new; they have been around for quite some time and have proven their worth in many business applications. Predictors, for instance, are excellent for forecasting numbers or categories based on historical data, while categorizers excel in sorting data into predefined groups. Both play a vital role in enhancing efficiency and decision-making in businesses, demonstrating that while generative AI is indeed captivating, it is not the most valuable AI player.
Practical Applications of Predictors and Categorizers
Predictors and categorizers serve as practical tools in various processes. Predictors are particularly handy when it comes to assisting decisions about future events based on past data. A great example of this is in predicting electricity usage, where the model takes into account factors like weather conditions, holidays, and user behavior to make accurate forecasts. On the other hand, categorizers are all about sorting information into specific groups. This can range from organizing emails based on their content to deciding if a customer qualifies for a discount. Both of these AI models play a crucial role in simplifying complex tasks and aiding in quicker, more informed decision-making processes in businesses.
Bringing AI to Business Users
The democratization of AI is not just vital, but indispensable. No longer should AI remain the exclusive domain of data scientists. Modern Generative AI (GenAI) tools provide simple user interfaces to generate text and images but we need the same simple interfaces for predictors and categorizers. These tools should enable business users to take full advantage of AI models without needing a deep understanding of the technical intricacies. The goal is to empower all members of a business to leverage AI in their daily tasks and decision-making processes, ensuring that the benefits of AI are distributed across the entire organization and not confined to just the technical departments.
Data Quality and Availability
Chris and I talked about how vital it is for businesses to have access to high-quality data when they are working with AI models. It’s not just about having a lot of data; the data must be clean, accurate, and relevant. This is because the AI models learn and make predictions based on this data. If the data quality is poor, it could lead AI to produce inaccurate predictions and potentially costly mistakes. So, businesses must invest time and resources in ensuring their data is of the highest quality before using it to train their AI models. This step is crucial for the successful implementation and performance of AI within any organization.
Practical Examples and Use Cases
There are a variety of practical examples and use cases where AI is making a significant impact on business processes. Integrating AI into daily workflows can streamline operations, enhance efficiency, and lead to better-informed decisions. For instance, AI can be used to sort through customer inquiries, categorize them, and ensure they get directed to the right department, saving time and improving customer service. Additionally, AI models can analyze sales data to predict future trends, helping businesses to better prepare and plan for what’s ahead. Incorporating AI into automated workflows benefits almost any department and function within a company. It’s about finding those areas where AI can make a meaningful difference and integrating the technology in a way that complements and enhances the existing processes.
Getting Starting with Predictors and Categorizers
When looking to implement AI, it’s crucial to first identify the processes within your operations that could truly benefit from predictors and categorizers. Once these areas are identified, the next step is to ensure that there is access to high-quality data, as this is foundational for the success of any AI initiative. Additionally, it’s important to involve business users in the implementation process. This not only helps in building trust in the AI models but also ensures that the tools are user-friendly and meet the actual needs of the business. By taking these thoughtful and strategic steps, businesses can set themselves up to successfully integrate AI into their operations.
Speakers
Transcription
Scott King
Hello everyone, I am Scott King and that is Chris Kraus. Thanks for joining us on this episode of the Union Podcast. We’re going to discuss AI, focusing on the hype versus the reality. The hype around generative AI, Chris, is significant, it’s tactically valuable, and people can use it.
However, there’s more value, there are more models in other types of AI. We’re going to talk about that – yes, there’s the hype and everyone’s trying to get on board, but there might be an easier and simpler opportunity you’re missing.
Chris Kraus
Exactly. We discussed this. It’s important to remember the types of AI that exist. There’s natural language processing and understanding. There’s categorizers that allow you to make decisions like categorizing an email. Is this email for a question on returns? Is this email a question on order statuses? Categorizing can also involve deciding from a list. Should this customer receive a discount? Yes or no, you’re choosing from a list.
There are also predictors, which might sound strange because you might think of predicting a category. But predictors are used when you’re predicting a number. An example John Michelson often gives is converting from Celsius to Fahrenheit. There’s a mathematical equation for that. But with a predictor in machine learning, you provide examples of both, and it determines what the temperature should be. Or if you want to predict, say, your electric consumption, electric companies do this. They consider the temperature, whether there’s a holiday in the week so you’ll be home versus at work to see if your usage patterns change. They predict what your electric consumption should be for your bill. These methods have been around a long time. They don’t have the hype of generative AI, which overshadows them. It’s raised our expectations. But there’s more we can do with machine learning models.
Chris Kraus
There are other forms of AI that provide great value, particularly to the enterprise.
Scott King
Indeed, John consistently emphasizes the value in predicting numbers and dates. They help guide financial decisions. We attended a generative AI summit. Oscar Marine, from ENY, agreed that predictors are highly valuable. Generative AI does hold some value. At a law firm, they discussed its use in generating contracts and completing paperwork. While time-saving, this doesn’t significantly impact the balance sheet.
Chris Kraus
Yes, that’s right.
Scott King
Let’s discuss predictors or categorizers. Could you explain what they are and how people are using them? I suspect it’s not as straightforward as it seems.
Chris Kraus
Certainly, the complexity depends on your perspective. There are numerous open-source, machine learning models. If you’re unfamiliar with the mathematics behind it, we’ve written a blog explaining AI and how machine learning works. I’ll keep it high-level here, but essentially, machine learning is a mathematical model. A predictor takes various numbers or attributes and predicts a number. For instance, your home’s electricity usage could be based on temperature or humidity, and whether you’re home or at work. You feed a machine learning model with historical data, and it starts predicting your electricity usage. If you supply it with data from the past year, including temperature, humidity, whether it was a weekday or holiday, and your electricity consumption, the model can then predict future usage based on this historical data and the given forecast.
Scott King
Can ERCOT utilize this? They seem to struggle with it.
Chris Kraus
Indeed, ERCOT and our local provider Encore do this. I received these predictions from my electric bill. They’re based on weather patterns.
As for categorizers, people understand this concept, particularly in the context of email. It’s about categorizing the email. For instance, the intent might be to answer a question about an invoice, a bill, or an FAQ. This could also apply to granting someone vacation leave. When a manager receives a vacation request, they consider various data. They’ll check the available vacation for the employee, the number of people on shift, and the warehouse’s activity level from the forecasting engine before granting or denying the leave. This involves a yes or no answer and a categorization.
Scott King
Unless your manager holds a grudge and adds bias to the equation, right?
Chris Kraus
Yes, the bias might be learned. There are many machine learning models. Textbooks on machine learning or websites discussing machine learning will tell you about pre-built, open-source models. These models contain the mathematical equations for number calculations and category predictions. There are different clustering analyses available. But to utilize these, you would need to become a data scientist. You might need to learn Python and start using tools like PyCharm and Kitlearn.
Scott King
Who is doing this? I assume if there’s an open-source model, it’s too technical for most internet users, right? They can use ChatGPT though.
Chris Kraus
Yes.
Scott King
It’s the machine learning with a friendly user interface. You’re discussing the models behind it. So, you need to be a data scientist. There’s a limited number of those individuals, right? We need to bridge the gap between what’s an open source model and how to make it usable.
Chris Kraus
And a programmer is required. A programmer can use them. A data scientist understands these models handle categorizations and clustering of data a specific way. The data scientist has the knowledge of, based on the problem, looking at the data, which model is needed.
Two models or three models could solve the problem. It’s a human process, a trial and error to find the right one. This accounts for 30% of the problems. There are pre-built models for these issues. Start applying and testing them. For the rest, there’s the multi-year data scientists.
They’re working to solve complex problems and write a new machine learning model. That’s another use case, the 30% one.
Scott King
The complicated ones equate to building businesses on top of AI products. We’re discussing helping everyday people make better decisions. We’re not talking about Netflix displaying the next title based on your viewing history.
Chris Kraus
Yes, absolutely. In this case, we need to pick the model based on the data. Ideally, clean data is available. A data scientist will analyze it for skewness, whether it’s time series data, and so on. They’ll then decide which model is the best fit and start testing them by writing code.
The challenge at the enterprise level is needing a technical person to handle this when you have hundreds of projects. We stack rank these on value, ask the data scientists about the level of effort to implement, and may need a team of data scientists to deploy them, test them, and bring them up, even though these are open source models. The question then becomes how to bring it to the customer and integrate it into an application or workflow for everyone to use.
People often get stuck at this point due to the many steps involved, which mirror the software development lifecycle.
Scott King
That sounds like it would take a while. Can you estimate how long the process you just described would take?
Chris Kraus
Software development life cycles tend to span three, six, or nine months when we do big room planning in Agile. It’s definitely a multi-month endeavor. However, at Krista, we believe there’s a better answer.
We’re aiming to address 30% of the problems with available open source models that can handle these calculations. The challenge is to have the machine do the work in choosing the right model and running the tests, essentially leveraging machine speed. This reduces the need for data scientists.
We’re not discussing those complex problems that require writing a model, but rather tasks learned in school, such as testing four models and discussing the differences.
Scott King
In that scenario, does this data scientist help with this project? We want to help them focus on the complicated models. This project that you’re discussing is less technical because the machine is doing all the testing. However, someone wanting to run this test may not have clean data. They say that in data science projects, the data is often the biggest problem due to required manipulation. What happens if I don’t have data?
Chris Kraus
The two problems you mentioned involve the audience. We want to simplify the process for business people so they can implement it without needing data scientists. That’s where we want the software to take over. We’re discussing machine learning and business people who understand their data. They can extract context and information from their emails and categorize them. They may have examples of data they can provide, such as decisions on customer discounts or electricity consumption. The closer these business people are to the data, the better they understand it. Not all data is perfect, but if you have 10,000 emails and three are miscategorized, it won’t skew the model. If 2% is miscategorized, it won’t distort the model. We trust that the model will learn from the more repeatable data. You either have some data to start with, or you have none. In the case of email triage, you likely have categorized sample emails. Humans categorized them, which may not be perfect.
Scott King
When you mention sample emails, Chris, I assume that could be extracted from a ticketing or CRM system?
Chris Kraus
Exactly, it’s possible to extract that data from systems like NetSuite or Salesforce. On the other hand, take the example of granting vacation. This process might be new, and while the data might not be readily available, that doesn’t mean the task is impossible. Ideally, we want the business personnel managing this process to train the model in real-time.
We could set up a workflow where an employee requests vacation for specific dates. In the background, the HR system checks the employee’s available vacation days. We could then look into success factors and identify the employee’s manager, determine the warehouse they’re working in, and during the requested time frame, we ask the forecasting engine about the expected busy period. We also check how many people are on shift using timesheets. All this data would be typically given to a human to make the decision. We’re essentially feeding the same data to the machine learning model to learn this decision-making process.
Chris Kraus
Initially, the AI model might not be confident of its answers, since it’s in learning mode. At this stage, the distinction between training and retraining is minimal; both processes are part of the same learning experience. We present a scenario to a human, without disclosing the AI’s answer, and ask them if they’d grant vacation based on the given information. The human’s answer then becomes training data for the machine learning model.
After a month or two, or after processing 200 or 300 such requests, the model can create a model automatically. It might run the data through multiple categorizers, such as random forests, perform clustering, and so forth. Initially, these predictions might have low confidence, especially if the data varies greatly, or they might have moderate confidence if the requests are similar.
This approach allows for training while in use, without the need for upfront training. It’s all about implementing your business process and letting the learning occur in the background. The challenge then becomes building users’ trust in the system.
Scott King
Indeed, the trust factor is significant. Managers usually go through this process, checking various systems and reaching a decision. The transition to machine-based decision making mimics this, but many unknowns can lead to a lack of trust.
Chris Kraus
Certainly.
Scott King
It’s captivating how this process could operate without internal data. It could function like eavesdropping, building data row by row in a table.
Chris Kraus
Absolutely.
Scott King
Despite this, there would still be a need for someone to finalize the process, making the ultimate decision.
Chris Kraus
Undoubtedly. The concept is to curate data and answers so that your well-qualified managers make decisions, after which the AI model learns to categorize these decisions. After a few months, the process can be modified slightly. For instance, the machine learning model can predict whether to grant a vacation or not, providing a confidence score for the prediction. Users will start seeing these decisions, comparing them with their own. Either they agree with the machine’s decision, or they disagree, which helps retrain and strengthen the model over time.
Chris Kraus
As the agreement increases, so does the trust in the AI. On the other hand, if there’s more disagreement, the model gets retrained and becomes stronger. If the AI consistently makes correct decisions, we can trust it and automate the process. It’s not a black box; users witness its improvement over time and notice its consistent agreement with predictions. The goal is to automate these processes, eliminating manual effort. We posit that there are hundreds of such processes in businesses that can be automated with machine learning models.
Scott King
We discussed the 30% example related to the vacation policy and email triage. Several of our customers have implemented this, saving a significant amount of time and effort. Can we provide other examples of this 30% improvement?
Chris Kraus
Certainly. One common scenario is when companies decide whether to offer a discount to retain a customer and prevent them from churning. If a customer is considering switching their streaming service, TV service, or phone company, the company might assess whether the customer is of high value and whether a discount might persuade them to stay. Instead of relegating this decision to a manager or a specially-qualified person, why not empower every customer service representative to make this call?
When I recently upgraded my iPhone, the company’s policy was to offer a new phone if the customer is halfway through their auto replacement plan. This is a rigid rule, but it could be more flexible depending on factors such as the size of the customer’s phone bill. Machine learning could allow for this flexibility, rather than strictly enforcing a rule based on time alone. For example, if a contract is more than 50% completed, the company might consider other factors in deciding whether to offer a new phone. This could add a level of nuance to the decision-making process.
Scott King
I’ve purchased items from this website several times. They have a rule that if you add something to your cart and close the browser, they immediately send a 5% discount for your cart. I use this strategy to get a 5% discount every time. The items are quite expensive, so it helps. The AI rules are a different game, but not as complex as people assume.
What do people need to start with? If they want to try one of these high volume, low risk situations with predictors and categorizers, what’s their first step?
Chris Kraus
Firstly, look at your business case. Is this something that happens every day, 100 times a day? You want to automate high volume tasks to get more value for your money. Secondly, consider the different input data needed to make a decision. Whether it’s predicting a number or categorizing something, you can contact us for help. The machine learning models are automatically built in the software. You provide us with an Excel or CSV file of the data and the expected answer.
We run it through the software and select a machine learning model for you. We’ll tell you how confident we are. The software trains on 80% of the data and tests on the remaining 20%. The question isn’t whether machine learning can help, but are you ready to make a change and is this a significant issue that happens frequently enough for you to want to automate it?
There are many machine learning models that can solve this. The challenge lies in operationalizing them and we can certainly assist with that.
Scott King
That makes sense, Chris. I appreciate it. “Am I ready for this?” might just be the title. Thank you for joining me for this episode of the Union Podcast, Chris. I enjoyed this episode. It was intriguing as it’s a bit outside of my field. Until next time.
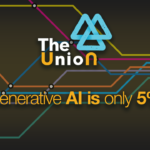
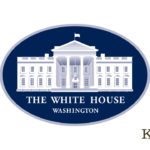
Read our comprehensive LLM testing guide
See how several LLMs fared in our test and steps to run your own tests for each of your use cases.
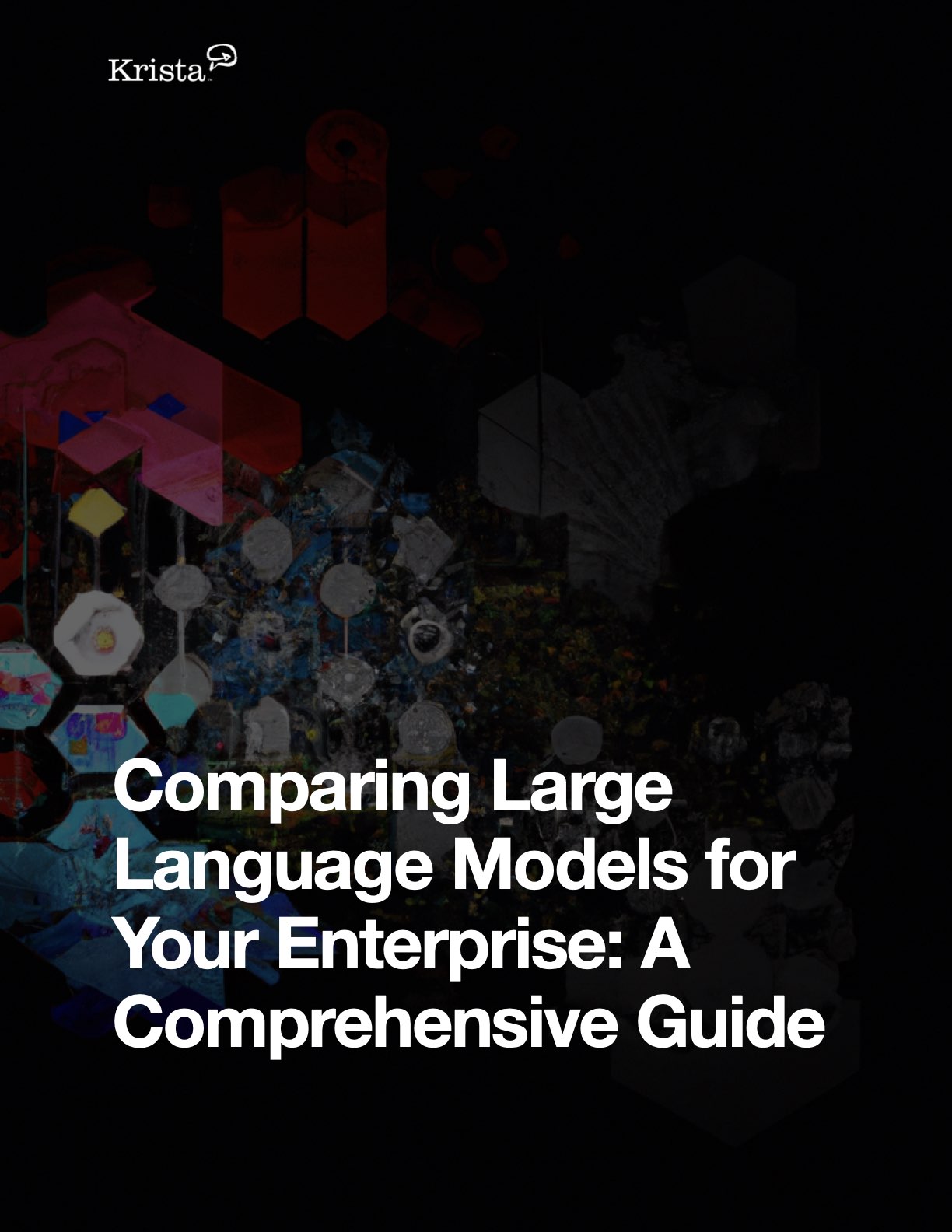