Is an AI Engineer Worth the Investment?
In an era where Artificial Intelligence (AI) is transforming business processes, companies face a significant dilemma: whether to hire expensive AI specialists or pivot towards more cost-effective and scalable options. With industry giants like Netflix offering compensation packages as high as $900,000 for AI Product Managers, the perceived value of AI expertise is high. However, smaller companies wanting to apply AI might be scared off by these numbers, raising questions about the viability and affordability of such roles. This article explores the financial implications of implementing AI, the challenges of AI adoption, and the benefits of choosing the right data platform for AI implementation. We delve into some AI use cases managed through automation versus those handled by specialized AI engineers, and describe the role of platforms like Krista in making AI accessible to companies regardless of their size.
The Rise of AI and Data Science Roles
The surging demand for AI and data science roles is undeniably shaping the modern job market landscape. High-profile companies such as Netflix have recently advertised positions for AI Product Managers with staggering compensation packages of up to $900,000. While this underscores the perceived value of AI within industry-leading corporations, it raises significant concerns for smaller companies aspiring to implement AI but unable to compete with such compensation packages. The feasibility of roles like these is also questionable. The high costs, combined with the limited available expertise, could lead to a situation where the demand for such specialized roles drastically outpaces the supply. Luther Birdzell points out, that many companies are attempting to approach AI in the same way as traditional software development. This approach does not account for the experimental nature of AI and the distinct need for automation, which could create a barrier to scale and continued accuracy. Therefore, it is important for organizations, regardless of size, to reevaluate their approach and understand the total cost of ownership before committing substantial capital to AI implementation.
Financial Considerations in AI Implementation
Companies, particularly smaller ones, may fear financial obligations tied to AI implementation given the exorbitant costs of hiring specialized AI talent as in the Netflix job post. Many companies may feel that their budget constraints might limit their ability to compete with industry giants able to offer lucrative compensation packages. The question then arises: Is the high investment in AI talent justifiable? Luther Birdzell points out an interesting perspective. He suggests that the traditional software development approach, which most companies tend to apply to AI, could be setting them up for a fall. AI, being highly experimental and requiring extensive automation, might lead to inefficiencies and financial drain if brute-forced through manual methods. Thus, before committing vast amounts of capital to hire AI specialists, companies should consider automation as a cost-effective and efficient alternative to conventional approaches. This might not only reduce the required investment in AI talent but also prevent the loss of critical time.
AI Adoption Challenges
Companies cannot simply buy their way out of the challenges that come with AI implementation. A common fallacy is that more AI use cases equate to more value and that high investment in hiring expensive AI talent can meet these demands. Comparing 1-2 AI use cases handled by specialized AI engineers versus 10 AI use cases managed through automation paints a different picture. In the former, extensive manual labor and vast amounts of capital are required, with the risk of inefficiency and drain on resources. But, when 10 AI use cases are managed through automation, the number of successful experiments increases significantly thus saving costs and time. It’s crucial to understand that automation does not eliminate the need for AI expertise, but it does make the expertise exponentially more effective and efficient. In essence, the successful implementation of AI is not only about the capital invested but also about the strategic and intelligent use of automation.
Efficiency with the Right Data Platform
Choosing the right data platform can significantly streamline the process of building and implementing AI models, offering a more efficient alternative to hiring an expensive AI engineer. A prime example of this is Krista, an AI-powered platform that allows companies to automate several types of use cases and add AI to processes. Krista’s capabilities facilitate experimentation with a wide array of AI use cases, thus leading to a considerable increase in successful experiments, time savings, and cost-effectiveness. Furthermore, the platform enables non-experts to integrate AI into processes without needing an expensive data science resource. Investing in an automation platform like Krista could provide a more practical, cost-effective solution for organizations seeking to harness the power of AI, rather than committing substantial capital to hiring specialized AI talent.
Investing in Cost-Effective AI
The escalating demand for AI talent, as exemplified by high compensation packages, has stirred concerns among smaller organizations aspiring for AI implementation. However, it’s crucial to understand that simply hiring expensive AI talent won’t solve the inherent challenges of AI adoption. AI is not a traditional software development task and requires a more experimental approach, with automation playing a pivotal role. Platforms like Krista offer a cost-effective and efficient alternative to hiring specialized AI talent, enabling companies to automate a variety of use cases and seamlessly integrate AI into their processes. Ultimately, companies should strategically leverage automation and pick the right data platform to ensure successful AI implementation, without unnecessarily committing vast capital to hire AI specialists.
Links and Resources
- The $900,000 AI Job is Here, The Wall Street Journal
- Technical Director, AI/ML, Netflix
Speakers
Transcription
Scott King
Hello, everyone. I’m Scott King, and joining me is Luther Birdsell, our chief AI officer at Krista. Thank you for tuning into this edition of the Union Podcast. Today’s discussion revolves around the demand for AI and data science skills within your data science team, whether you already have one, or are considering establishing one.
Luther Birdzell
I’m doing well, Scott. It’s great to be here.
Scott King
A point of discussion is the need for a $900,000 AI engineer. This idea comes from a Netflix job posting for an AI product manager with a salary range of $300,000 to $900,000. This includes stock options and benefit payments, but $900,000 is a considerable amount. Luther, is such an expensive AI engineer necessary?
Luther Birdzell
Scott, it would be an exceptional circumstance for an organization to find that such a payroll commitment yields maximum shareholder value. It’s not impossible, but it’s likely for a small minority of cases.
Scott King
Many businesses want to integrate some form of AI, whether it’s generative AI in the sales and marketing department, or predictive analytics for forecasting or cash flow analysis. However, the large salary of a $900,000 AI engineer can deter them as not everyone can afford this.
Luther Birdzell
Scott, this is classic innovator’s dilemma. The really big companies with enormous balance sheets have made exorbitant financial commitments early in other technology cycles and not always emerged victorious. These resources and other dynamics often get used as we move away from Fortune 500 and Global 2000 into the middle market, especially the lower middle market, and beyond into smaller businesses. As we move down that spectrum, these options become more limited. This situation has always forced small businesses to be more creative in resourcing any kind of problem, particularly technology.
That’s the essence of innovation where the smaller, agile companies can have an advantage. Almost everyone needs AI and a building sentiment to remain competitive. My ice cream shop, and my barber, don’t require it as soon as some of these bigger companies. But if we just focus on medium-sized businesses, companies with revenue maybe over $5 million, and then onto the biggest companies in the world, there’s an increasing imperative that no one wants to be only talking about AI in January of ’24. If you’re not doing something when the calendar year changes, there is a consensus building that these are the people who are behind.
Your typical technology cycle, ‘Crossing the Chasm‘, lays this out nicely. You’ve got your visionaries, the first to adopt, then your early adopters, and crossing the chasm is starting to sell to the mainstream. You get a large mainstream population, and then you get the laggards. AI is presenting, as John says, you can be early or you can be late. AI is becoming increasingly binary on the adoption spectrum. You’re going to be off the mark doing something and in the group that’s moving towards a lead, or you’re a laggard. Waiting for the mainstream, de-risking our investment by letting everyone else figure this out first, is looking less and less like a successful strategy in AI. In a short answer, Scott. Yes, there is increasing pressure to be doing something with AI now or very soon.
Scott King
If people see the expensive engineering job, they may be scared off. They might think they can’t do it. But if they don’t have a big budget and they want to get into AI, what do they do? Many of our customers don’t have data science teams. Or if they do, it’s in a completely other part of the business, not on the automation side. What do they do? From today until January, if you’re not doing AI, what do you do? Do you try this in a low-risk situation? Do you hire somebody to help you figure it out? What should people do?
Luther Birdzell
Scott, just because a company can try to brute force AI with high priced resources doesn’t mean they should. That’s the first thing. The answer to the question applies to everyone, whether they have the option to deploy a lot of money to hire many data science teams or not, is to start with the right strategy. What I’ve seen in the market over the past decade is that when organizations focus on automating the learning process, instead of getting distracted by using complex technology to automate human tasks, there’s a big differentiator there. It might seem like just semantics on the surface. Let me walk through an example.
Scott King
What do you mean by automate the learning process?
Luther Birdzell
I’ll provide an example. Whether we’re a medium or a large company, a data science team, whether they’re our employees or outsourced, is going to have to set this up. And whether we’re using large language models or traditional machine learning, there’s some kind of data that we are going to present to some kind of machine learning or AI model. That’s the input. We’re going to get some kind of outputs from this.
An output might be predicting a number, telling me what something is, it could be a summary of a document, or many other things, especially under that large language model, but we’ll get to that. You need some folks to set this up. Once it’s set up, the default pattern in organizations is that data science team then needs to basically stay married to that project to continue to update, to improve it, or even just keep it as accurate as it already is.
This means you then need to hire a second data science team to do project two which basically gets entrenched in that, and a third one to do three. Only the big companies can really afford to do that when those data science teams cost typically one to two million annually or more if you’re trying to grab premium resources.
Very few companies even have the option to do that. When you compare this to one data science team starting the first project, then move on to the second project, and every once in a while, maybe once a week, they get a notification from the system that we’ve updated the model. Using the same pipelines and everything that they used to set up project one, we’ve updated that model, it’s scoring better than the model that’s currently in production.
They have their reports and all the stuff that they set up to help them compare the models on the path to the first production deployment. They can then look at that and we bring a human in the loop to apply that expert judgment that takes 10 to 15 minutes. Then they can either say, keep working on this and let me know next time you think you’ve got something better or press the other button and it automatically updates what’s in production.
One data science team can now do 10 projects, deliver, maintain, and continue to optimize 10 projects instead of needing 10 teams to do those 10 projects. The cost basis is wildly different. Big companies who try to brute force that are likely to get passed by medium-sized companies generating a lot more velocity.
Regular software involves humans writing and updating code. We introduced machine learning, which is some of the code, the really valuable, tricky logic. That’s machine-written code, but by default that’s updated manually. That gets really expensive. That’s the model where you need a data science team to both setup and then own and stay attached to each project that you do.
When you can automate the process of updating the computer-generated code, you’ve got an organization that’s learning at automated machine speed, and a company that is learning at manual human speed is not going to be able to compete with a company that’s learning at automated machine speed for long. The cost basis is completely different, but it also results in a competitive acceleration to the improvement of the business and the learning in that business.
A big company doing it manually can’t compete with a much smaller company doing it in an automated way. That’s where the value is in AI. AI is the first technology in the history of computing that has the potential to really learn on its own. When we can tap into that, that’s what’s special about AI. We often hear about AI automating human tasks.
The IBM AS360 in the 1960s automated arithmetic and changed the world. And software, as it’s continued to improve and computing’s improved and we’ve gotten better at writing the software, we just automate increasingly sophisticated human tasks. But that’s what software has always done. The unique special value-driving part of AI is when we can automate the learning process. That’s what changes everything.
Luther Birdzell
If you bring someone in who can automate the learning process and enable a small team to achieve a lot, you have a valuable resource. It’s also a scarce resource. For some organizations, bringing in that kind of expertise could yield a significant return on a small team of potentially expensive people. There are AI-focused orchestration platforms in the market today that understand the inputs and outputs to these systems. This understanding is crucial in every regulated industry because it forms an audit record. The same data can then be used to automate the learning process of AI-centric solutions.
Some people may choose to build this technology themselves, which may seem appealing. We have customers who’ve reallocated over $100 million of budget to a more self-learning strategy, deviating from the manual one they were planning to scale. If you bring in technology that allows you to get more done faster, potentially even enabling power users to participate more in the process of connecting the systems in the business with the data in the business with the people and the AI, then your engineers can focus exclusively on creating the kind of intellectual property that creates real competitive differentiation. The first companies to do this are in the strongest position to build truly sustainable competitive advantage and true moats around their AI technology. These are the large companies that will stay large and become larger. These are also the medium companies that are going to displace some of the current big ones and become big themselves.
Scott King
Indeed, or they become big themselves until a larger company with a larger budget acquires the competitor, right? Because they’ve gained so much market share and started with the automated learning process. That’s why software companies get gobbled up.
Luther Birdzell
Absolutely, a medium business just created massive shareholder value as the bigger company will pay a considerable premium to acquire that technology. That’s a significant win for the shareholders of the organization that adopted, committed to, and executed a real AI strategy centered around automated learning.
Scott King
Shifting gears, a recent article in the Wall Street Journal reported employee churn in AI companies, including data scientists. Why do you think that is? Is it due to unsustainable hype and cost structures? It seems paradoxical that while everyone’s buying AI, the AI companies are laying off staff.
Luther Birdzell
From a distance, I agree, it seems paradoxical. But considering the speculative bubble dynamics that shift their focus from one thing to another, it starts to make sense. The fundamental problem with AI is that the way most people approach it is too expensive to create real value for most businesses.
They attempt AI in the same way as traditional software development, ignoring the fact that software was humans explicitly programming rules into a computer, creating a complexity ceiling. Everything was always manually updated, typically on an iterative basis. The difference with AI is that it’s highly experimental, running hundreds of thousands, even millions of experiments.
Many succeeded in building great software without using automation. However, few are delivering high-value AI without heavily leveraging automation due to the sheer number of experiments needed. This is just the setup; to keep it in production and maintain accuracy requires continuous work that no one can keep up with manually, no matter how much money they invest.
We must automate much of the experimental process. That creates a lot of value but is not the way most are approaching or talking about AI. It hasn’t been the starting point for a single customer conversation I’ve had this year.
Scott King
Powerful. I appreciate it, Luther. Thanks for walking through this story. I found it interesting because I thought if somebody’s going to pay that much for a person, the little guy is never going to be able to afford that. But they’re still going to be doing something. They have to figure out a different cost model to deploy AI. Any last thoughts? Anything you want to leave us with?
Luther Birdzell
Scott, I’d advise anyone, any size organization, before they commit capital, especially before they commit a lot of capital, to thoroughly understand not only the cost to get to production but also the cost of ownership. The cost of ownership of AI is much higher than traditional software, and organizations that think they’re going to be successful with AI by doing it exactly the same way that they did traditional software are not only spending a lot of money, but they’re also losing critical time. That’s the word of caution. Before you try to manually brute force an AI implementation, make sure you understand how the role of automation, or the lack thereof, is going to impact the total cost of ownership of that project.
Scott King
Okay, I appreciate it. Thanks, Luther, for talking to me on this episode of the Union Podcast, and I’ll check in with you next time.
Luther Birdzell
Alright, thanks, Scott. See you soon.
Read our comprehensive LLM testing guide
See how several LLMs fared in our test and steps to run your own tests for each of your use cases.
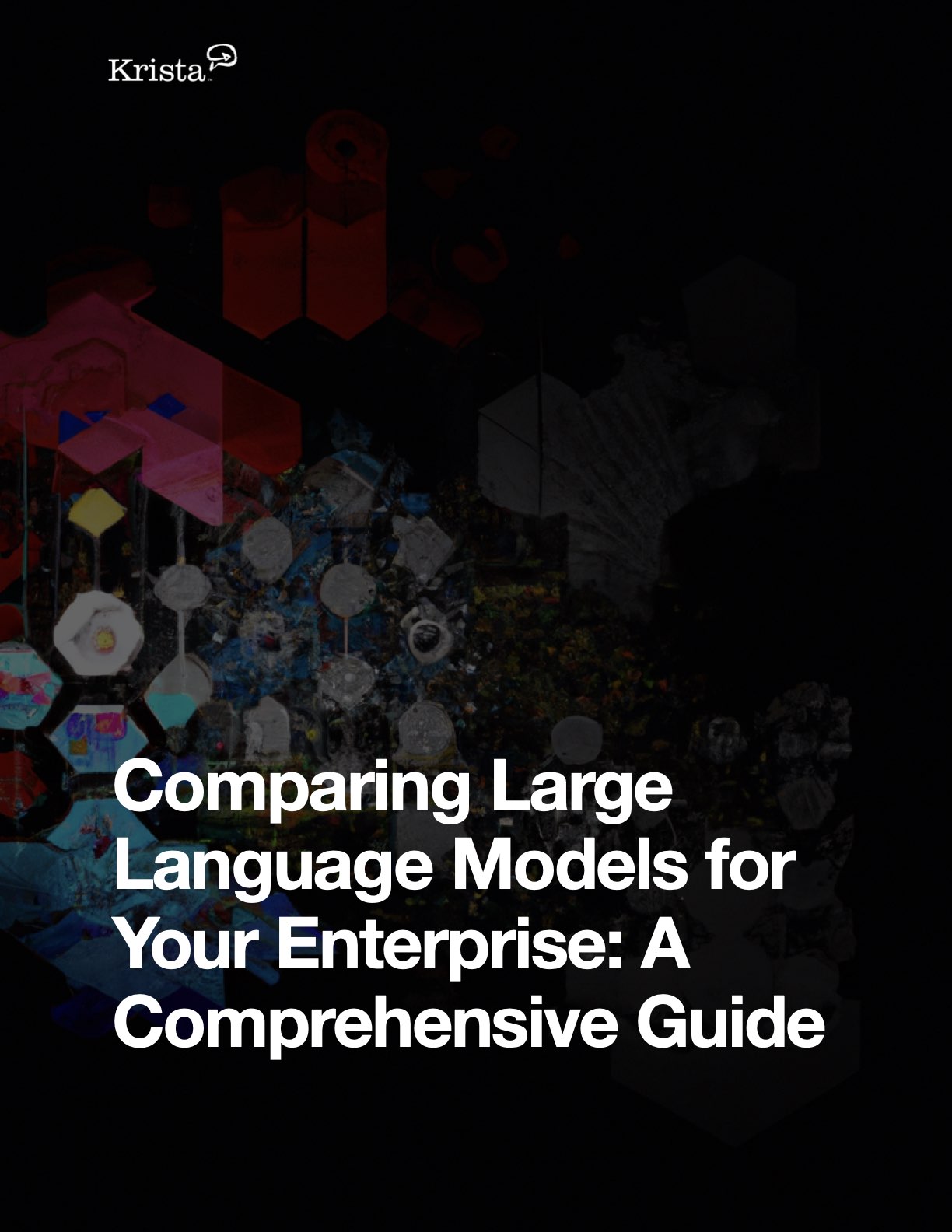