Companies evaluating AI want the most accurate machine learning models (ML) to predict or summarize business outcomes. However, many times achieving high levels of accuracy and confidence inflates expenses and erodes value versus adding to it. In this episode, Luther and I discuss what is and what is not AI, evaluating ML model accuracy against costs, and how to maximize your ROI when building or maintaining your models.
What is AI and What is Not AI?
AI, or Artificial Intelligence, is a concept that is often misunderstood or mislabeled. To provide some clarity, there are essentially three classes of software in the market today that people are calling AI.
The first class is merely regular software which is inherently rules-based. Humans write the code, and it is manually updated. Despite the prevalence of this software being called AI, it is just ordinary software. If humans are writing and updating the code, it’s just regular software, as we’ve always known.
The second class, which is one step up the maturity curve, is what we refer to as naive AI. This is when machine learning is introduced into traditional software. In this case, machines study historical data and write some of the code. However, the system is still primarily updated manually. Manual updates in this class contribute significantly to why the global return on investment in AI ranges between just 2% and 11%.
The third class is what we consider real AI—the technology that can genuinely transform markets at scale. In this case, machines are writing more of the code, although not necessarily all of it. The key differentiator here is that the learning process is automated. However, it’s important to note that this does not mean that there’s no governance, or that the computers autonomously replace what’s in production with something they think might be better from day one.
How to be successful with AI
The key to success with AI lies in the optimization of resources and costs, achieved through the automation of the learning process. In the context of real AI—the technology that can genuinely transform markets at scale—machines are assuming greater responsibility in writing the code. This does not eliminate the necessity of governance, nor does it suggest that computers autonomously supplant existing systems from the onset. Rather, it implies a shift in the role of data science teams, who traditionally execute the first phase of a project and then expand to maintain the system at a significant cost. With automation, the need for manual updates and the requirement for multiple dedicated project teams can be replaced by a solitary, versatile data science team. This team not only executes the initial project but can also focus on subsequent projects, dedicating a fraction of their time to evaluating new models automatically developed by the system. The AI, in essence, builds and suggests more accurate models that are vetted by human experts before being implemented. This real AI model can create a high return on investment by reducing the cost of ownership significantly below that of staffing and maintaining a data science team for every project. This approach is more viable as fewer companies have business models that can uphold such cost structures for these technologies. Hence, successful AI implementation involves a strategic shift from manual work to automation, democratizing authorship and elevating manual workers to reviewers—an approach that fundamentally automates learning, delivering substantial value.
Evaluating Machine Learning Model Accuracy
When it comes to evaluating machine learning model accuracy, the balance between costs and benefits plays a crucial role. The primary goal is to generate value at scale and do so consistently, which is the real measure of success with AI and software. When considering the investment in AI, the first key threshold to identify is the break-even point—how much money must be spent to realize a return on the technology. If the investment is lower than this point, the AI’s accuracy may be compromised, potentially leading to value destruction. Therefore, determining the initial accuracy threshold, at which AI can positively contribute to the business economically, is essential.
Without this analysis, businesses run the risk of letting emotional rationalizations guide their decision-making process. For instance, they might insist on a 90-95% accuracy threshold for implementing machine learning or AI, even when the current manual process might only be 60% accurate. This mindset not only overlooks the existing baseline but also reality, leading to excessive spending in pursuit of an unattainable accuracy level.
Moreover, the cost curve in relation to accuracy is highly nonlinear. For instance, the cost could be $100,000 for a 50% accurate model, $500,000 for 60% accuracy, and $1,000,000 for 70% accuracy. To reach 90 or 95% accuracy, the cost could balloon to $10 million or even $100 million. This depicts the steep, nonlinear cost curve associated with chasing high precision, which many problems don’t require. Therefore, it is more economical to focus on reaching the minimum investment threshold to break even, ideally surpassing it slightly in phase one, to gain positive value contributions through cost savings and value creation. This approach allows businesses to benefit from machine learning in a cost-effective and efficient manner, potentially achieving an average ROI that is multiple times higher.
How to balance ML budgets for the highest ROI
To strike a balance between machine learning budgets and accuracy for the highest ROI, it’s crucial to incorporate cost data into the value creation or cost-saving equation at varying accuracy thresholds or automation levels. It is a complex process, and by avoiding it, many are likely hampering their own success. For instance, if we were to automate a task with a model that gets 172 out of the 316 predictions wrong, we would end up destroying value instead of creating it. We need to hit a 60% accuracy level, where we’re correct enough times to create roughly a million dollars of value. If we can achieve this for an investment of less than a million, ideally less than half a million, then we’re looking at a multiple X-ROI. This would significantly outperform the market by a hundredfold.
Such an analysis helps determine feasible projects. If we believe an investment of less than a million dollars, particularly under half a million, can be achieved, we should proceed. While we continue to invest in increasing accuracy, we need to maintain a focus on cost-effectiveness. It’s essential to remember that we don’t need to wait until we have achieved 90% accuracy before implementing.
The financial analysis shows that we need to be over 50% accurate, but not necessarily reach 60%, to outperform the market with AI by 100X. This critical insight is often overlooked. As we initiate the project and refine the cost, we gain a clearer idea of what it would take to increase accuracy from 60% to 70%, 80%, and eventually 90%.
Inclining towards excessive spending to brute-force accuracy not only consumes more resources than necessary but may also extend the time required to achieve the objective. It comes at the expense of other potential initiatives that could also create significant value at initial accuracy thresholds of 60% or 70%.
As we continue to invest and enable automated learning, these models will self-improve from 60% to 70% and 80% with minimal human intervention, as long as the system is being actively utilized. The more the system is used, the more data it amasses, allowing internal AI to train new models and present those with higher accuracy than the current models.
A deep focus on financial analysis both before the project’s commencement and throughout its duration is key to producing optimal results. This has been true for traditional software and is even more crucial for AI, where overspending and the need for a careful balance between depth and breadth of use cases are critical to creating value for the organization at scale consistently.
How to get started with machine learning
The strategic implementation of AI and machine learning can deliver substantial value to businesses by automating learning and efficiently shifting from manual work to automation. However, the key is to achieve a balance between the costs and benefits. It’s crucial to avoid chasing an unrealistic accuracy threshold, which could lead to excessive spending and potential value destruction. Instead, businesses should aim for reaching an initial accuracy threshold to which AI can economically contribute value. This involves an in-depth financial analysis to identify feasible projects and avoid hampering success. Remember, the more the AI system is used, the more data it collects, improving its accuracy over time. Therefore, balancing spend and focusing on cost-effective implementation is vital for consistent value creation at scale with AI.
Links and Resources
Speakers
Transcription
Scott King:
All right, good. All right, well, hey, everyone. I am Scott King, and I’m joined today by our chief data scientist at Krista Luther Birdzell. Luther, how are you?
Luther Birdzell:
Hey, very well, Scott. Great to be here.
Scott King:
Hey, glad to have you. Today we’re gonna talk about how to intelligently invest dollars and data into machine learning and AI. Luther’s going to tell us how to get the most out of our budget, some steps that we can go through to evaluate what’s real AI, how to look at it, and how to understand the quality of responses and the trust in AI. Luther, can you give us a little bit, just a brief overview of maybe what everybody’s going to learn today? And then we’ll kind of get into kind of level setting what real AI is.
Luther Birdzell:
Great, thanks, Scott. So, the general theme here is time-tested best practices from traditional software that are not being applied to AI, to the detriment of the AI projects. The first theme, the underlying theme here, is automation. A lot of folks create a lot of great software without having to automate a lot of the software development process. Now, some of us who have actually built businesses around software automation, are deeply familiar with the value that software automation can contribute. But again, historically, a lot of projects didn’t need it. With AI, what we’re seeing is that not only are the pieces of automation critical, but automating the entire process, such that the learning can become automated, is really critical for the economics. And we’re going to unpack that here. So, the broad theme is traditional best practices for software applied to AI. Specifically, this one is automation as well, and we’re going to go, follow the money. We’re going to unpack the dollars around why the automation is valuable and really take a look at that economic analysis, which kind of leads into another best practice, Scott. Historically, half of traditional software projects have failed by the highest standard of delivering on time and on budget, essentially delivering the value that rationalized the budget to start the project. In my experience over the past 25 years, Scott, the projects that model the economics, set economic goals, and then manage to those economic goals throughout the software project are disproportionately in the winner circle, in that half of projects that are delivering on time on budget. Very, very few AI projects have any real financial modeling behind them. And it’s tricky. It’s a new skill that I really consider to be part of executive financial literacy, but it requires more technical depth than a lot of corporate financial modeling has up to this point. So, that’s really what we’re going to dig into and take a look at.
Scott King:
Alright, well, that makes sense because everyone who listens here has delivered software and built software. However, software is never truly done, right? You’re always working on it. I think you’re alluding to scope creep, and perhaps even agility. So, if people claim that they do numerous sprints and they’re agile, I believe what we’re going to learn today is that we need to redefine what “agile” really means. Learning occurs much faster. So, what are some different aspects of this? I know we discussed what real AI is and what it isn’t.
What is AI and what is not AI?
Luther Birdzell:
Absolutely. So Scott, the simplest explanation I can provide is that there are essentially three classes of AI in the market today. The first is inherently rules-based, regular software. Humans write the code, and it is manually updated. Realistically, there’s nothing AI about this, except that most of the software that folks are calling AI is just ordinary software. It’s significant to note that this is not what we’re focusing on here. It’s essential to be able to differentiate this. Fundamentally, if humans are writing and updating the code, it’s just regular software, as we’ve always known.
The next step up that maturity curve is when we introduce machine learning into traditional software. In this case, machines study historical data and write some of the code. However, the system is still primarily updated manually. We refer to this as naive AI. When we examine why the average global return on investment in AI ranges between 2% and 11%, these manual updates contribute significantly.
As we consider real AI—the technology that can genuinely transform markets at scale—machines are writing more of the code, although not necessarily all of it. But the substantial differentiator here is that we’re automating the learning process. I want to clarify here, Scott, this does not mean that there’s no governance or that the computers, from day one, are autonomously replacing what’s currently in production with something they think might be better.
What it implies is that instead of having a data science team perform phase one of the project and then typically expand that data science team (which, on average, incurs at least a million dollars a year of carrying cost) just to maintain the first project and then staff another team to initiate the second project—you can see how that gets expensive rather quickly. On the one hand, if we’re manually updating this, that is the model, and that is the status quo. It contributes significantly to why there’s such a low return on investment in AI up to this point.
The alternative is to have one data science team. They execute the first project, but we automate the learning process. Therefore, instead of having those data scientists and software engineers collaborating to manually continue updating the system, data science team one can start working on project two, perhaps dedicating five to ten percent of their time to evaluating models that are automatically built by the system using the new data flowing through it.
When the software and the AI—this is really AI to implement AI—believes that it has trained new models with new data that are more accurate than what’s in production, it presents those models to a human data scientist or subject matter expert for evaluation before it replaces what’s in production.
Scott, when we think about our broader theme at Krista of enabling a shift in the author dynamic—essentially democratizing that authorship, moving people away from manual work and relying more heavily on automation, and elevating those who are in the trenches doing all this manual work to the reviewer level—we all have the capacity to review a lot more than we can actually create in the same business day.
Fundamentally, real AI automates learning. That’s the key difference. That’s where the real value is with AI. So when we think about it, all software automates human tasks. Most of it’s expensive to create, but to consistently deliver a high return on investment, you need to reduce the cost of ownership well below the cost of hiring and maintaining a data science team for every project that a company desires to undertake in-house. Again, this is because so few companies have business models that support such a cost structure for this technology.
Scott King:
I imagine that’s how people would get started, right? They would look at that first project, then understand the maintenance requirements if they didn’t automate the learning process like you were talking about. If they didn’t do that, and then they did hire, or even sought to hire, right? Because I think the demand for the data scientist role is going to outpace the capacity to supply that demand. So, those guys and gals just aren’t going to be available, right? And if they are, the cost increases. So, you have to do it the other way around. Let’s get into some of the cost benefits. How do we do this? What’s the first step in looking at delivering that value, and working towards the automated learning process?
Luther Birdzell:
First and foremost, organizations must abandon the notion that achieving success with traditional software ensures similar triumph with AI using identical resources and strategies. This erroneous assumption is the root of many issues. Recognizing AI as a distinct entity, it is clear that we must approach it differently from traditional software to achieve success. Furthermore, in a landscape where only a handful of companies are creating substantial value, there is a necessity to deviate from the status quo in order to outpace the market.
Scott King:
I understand it’s a cliché to say I want to “double click”, but let’s explore your statement further. As an IT leader, what should make me believe that I have succeeded in delivering software? What creates the assumption that I can also tackle AI? Can you provide more details about your point?
What makes someone think they will be successful with AI?
Luther Birdzell:
Sure, Scott. There are two ways to gauge success with software. One of them aligns with the standards of AI, which I believe should be the benchmark for all technology and, frankly, all business investments. The question is, did you make the business more valuable? Fundamentally, this is the reason to invest in a business – to increase its value. So, whether the investment is in software, AI, or new trucks for the fleet, are we maximizing the return on the capital that we invested to enhance the business value? If the software achieved that, great, it’s a success. However, not all organizations hold their software or technology investments to this standard, at least not consistently throughout the organization. The other perspective is whether the software worked. Or, did the project get canceled before it consumed too much time and money, leading to its termination by those who were to receive it? I believe following the money and applying rigorous economic standards are the ways to measure success, as this approach normalizes the investment across any alternative. Nevertheless, some still attempt to skirt by on lower standards, Scott. Their basic criteria are, did the thing work? Did I avoid getting fired for participating in or managing the project? These are really low bars for defining success. And it’s not unique to software; it applies to any initiatives within companies. When we, in the absent financial discipline, translate whatever it is that we’re doing into the language of the business, the dollars and cents, look, we make up all kinds of crazy rational… We rationalize failure. as success in all kinds of crazy ways.
Scott King:
Yeah, yeah. I mean… The answer is going to be different for everybody, right? But, you know, I just want to make sure that everyone understands that, like, this is not easy, right? So, you know, because you’re talking about big budget dollars and big projects, and, you know, the risk level is lower and higher, depending on where you get started. But I just wanted to make sure and really discover that, hey, this is tough, right? The not get fired is, I didn’t expect you to say that, yeah, it’s a pretty low bar. We’re not gonna use that, but let’s follow the money.
Luther Birdzell:
Okay, good. So, that’s the high bar, really. That’s the old standard, you know? Were we successful? Did we create value at scale? Can we do that consistently? That’s really being successful with software. So the first step, and you know, again, being specific to AI here, although a lot of this does generalize to any kind of investing, is that if we look at our value analysis cost benefit slide, and this is kind of starting at the end of the story, there are a couple of key thresholds, Scott, that we want to identify.
The first is, at what investment, at what cost – like how much money do we need to spend, basically to break even on the technology? If we spend less than that and we go and use this AI, it’s not going to be accurate enough. And it can actually destroy value. It can destroy a lot of value very quickly. So, it’s really important to know kind of where that initial threshold is. This is the initial accuracy threshold at which machine learning and AI can start making a positive economic contribution to the business.
And Scott, absent this analysis, I have seen over, and over, and over again, the emotional rationalization. Of this is an important business, this is an important decision, we wouldn’t possibly consider using machine learning or AI that’s not at least 90 or 95 percent accurate. Their baseline on accuracy in that decision that’s made manually today might be 60 percent. It might cost an inordinate amount of money to arrive at all of those 60% of accurate answers, and all those 40% inaccurate ones. So, not only is it disconnected from the baseline, but it’s really just fully disconnected from reality.
What they wind up doing is spending a huge amount of money charging right past the points where the spending threshold, the investment threshold, which they would have really created value for the business, and spending so much, chasing accuracy that they don’t have today and that they don’t need anything near to create real value for the business. And they just wind up way overspending.
And the piece to consider there is, that the cost curve is very nonlinear as it relates to accuracy. So, I’m just going to use some round numbers. If it costs like 100 grand to get a model that’s like 50% accurate, and like 500 grand to get to 60 and maybe a million to get to 70. It can become 10 million, or sometimes even 100 million to get to 90 or 95%. So you get that steep nonlinear cost curve chasing that high precision, which a lot of problems don’t even support at all, at the expense of knowing where the minimum investment threshold to break even, ideally going a little bit past that in phase one, is getting that positive value contribution, both in cost savings and value creation, deployed to production at that economic threshold.
And we follow the money. It really keeps our emotions out of this. There’s so many businesses that can benefit so much from machine learning that’s relatively easy and relatively inexpensive to get started with, that can create not just single digit ROI, but actually have a multiple of average ROI.
Scott King:
Yes, that is indeed an important point. We’ve previously discussed on the podcast about the topic of trust in AI. People desire high confidence levels because they want to trust AI. However, it should be noted that even humans performing the job aren’t 100% accurate. Human error is present everywhere. These humans don’t cost 10 million dollars unlike a machine learning model that could be 95% accurate or thereabouts. So, how do people judge where there is under-investment or over-investment? What is the method to determine this?
How do you determine how much to spend on your machine learning model?
Luther Birdzell:
Sure. So, Scott, the next point on this slide says, “Model Performance Report and Step One”. The data science team is going to utilize the organization’s historical data for this step. They’re going to experiment with different configurations of this data, try different algorithms, and train various models. Subsequently, they are going to identify some models that perform better than others. Generally, it’s the average performance of these models that we’re going to use to select the best average performing model. Furthermore, we’re going to quantify how much that model gets wrong and how much it gets right using data that the model has never seen before.
When the data scientists start training the model, they typically use about 80% of the data. This percentage can vary depending on the data set size. Generally, you train the models on 80%, and then you do blind testing with about the other 20%. So, the blind testing allows us to see what we get right and what we get wrong. The data scientists usually send color-coded reports, but it’s at this point that the disconnect starts. This is where new learning, especially at the executive level, has to take place for economic modeling of AI to become more mainstream.
In this color-coded report, dark blue indicates good performance, white is so-so, and red is bad. While this gives an intuitive feeling, it’s the next step where the rubber really meets the road. There are three technical steps needed to translate the data scientist’s report on the model’s performance, the blind testing results, and the actual economics of the business.
This process starts with a baseline. Typically, if AI is involved, especially if we are involved with AI, we’re automating something. The first thing we need to establish is how much it costs to do that task manually today. In our example here in step number one, “Baseline in the economics”, it’s important to consider how many people are needed, how much we pay them, and how long it takes them to do the task.
For instance, let’s imagine we have a team of 50. This could be an onshore or offshore team. We account for the team size and the hourly labor cost, which includes health insurance, payroll tax, and other related costs. In our example, it takes a human approximately 10 minutes to complete this task. Therefore, simple arithmetic indicates that the cost per action is $16.67.
This calculation allows us to determine potential cost savings or cost avoidance. For every task that the AI accurately automates, we can claim a cost saving of $16.67. However, when the AI makes an incorrect prediction, the cost is higher than if the AI hadn’t been involved at all, as a human now has to find and fix the problem, then do it manually as they would have if the AI had never been involved.
In the bottom right-hand corner of the model value chart, the cost of incorrect predictions is represented by two yellow columns. If an action costs us $10 to do right, when the AI is wrong, we are penalized $15. That translates to a 150% cost.
Scott King:
Okay, yeah, so in our example, the cost per action is, I’ll call it 16, so it’s 24 dollars.
Luther Birdzell:
Exactly. Yep. So we get credit for $16 if we’re right, and we are subtracted, we are penalized $24 every time we’re wrong.
Scott King:
What is that? Is the 150 percent? Is that industry?
Luther Birdzell:
Scott, this was specific to the customer we were working with concerning Krista’s AI email readers. These are real numbers from a call center that employs 50 people in the US for advanced support. It’s quite expensive. They’re constantly reading customer requests for service or support related to their products, and they spend around $10 million a year on this.
Scott King:
Okay. Can you explain how you calculate the graph’s ‘Translate Model Performance’ into annual savings?
Luther Birdzell:
Sure, Scott. The first step was to establish a baseline, simple arithmetic. We’ve covered the part about the extra penalty for being incorrect. Now, we move to the technical translation. Here we consider different model accuracy thresholds. ‘Prediction confidence’ is how accurate the model thinks it is, while ‘percent correct’ shows how accurate the model actually is. We validate this by using real data from the model in a blind test set of 400 emails. In this case, if the model thinks it’s going to get 90% right, there are 219 out of 400 emails that fall into this category. So, as the prediction confidence decreases, the number of predictions increases. However, we also see that we get 205 out of 219 right at 90%. Therefore, there’s a lot of positive contribution and very little negative contribution. That’s why with an extremely accurate model, we could potentially automate about 8.5 million dollars of the 10 million dollars. But what’s not considered here yet is: how much does it cost us to achieve this?
Scott King:
That leads me to my next question. If I understand correctly, this is a customer service example. Say, I’m getting 80% model confidence. As a leader in customer service, I’m interested in two things: beginning a different project or enhancing the accuracy of my model. With my resources, I need to decide which one to pursue. How do I make that choice? Also, how can I determine what the optimal level of confidence is?
Luther Birdzell:
Scott, the straightforward answer is that you need to incorporate the cost data into the value creation or cost saving at various accuracy thresholds or automation levels. It’s not easy, we established that from the start. Most people aren’t doing this because it’s complicated, and they’re likely harming themselves by not doing it. We can quickly determine that if we automate half of this with this model and get 172 out of the 316 wrong, we’ll destroy value. We don’t want that.
At a 60% accuracy level, we’re getting enough right to create about a million dollars of value. Therefore, if we can create a million dollars of value for less than a million-dollar investment, preferably less than half a million, we’re looking at a multiple X-ROI. This ROI is vastly outperforming the market — the market gives single-digit percent, and we’re getting single-digit X, outperforming by an impressive 100X.
From this analysis, we can ascertain that if we believe we can achieve less than 500 grand, especially less than a million, we should proceed. We should estimate the cost, assess how we can do it more cost-effectively as we continue to invest in increasing accuracy. However, we should emphasize that we’re not going to wait until we’re 90% accurate before going into production.
We’ve followed the money, and it suggests that we need to be over 50% accurate but don’t need to reach 60% to significantly outperform the market with AI by 100X. This crucial detail is overlooked without this analysis.
We begin the project, refine the cost, optimize it, and get a clear picture of how much it will cost to move from 60% to 70%, to 80%, and eventually to 90%. We aspire to reach there, but if we spend excessive money trying to brute-force it, we’ll not only spend more than necessary, but it will also probably take longer.
Moreover, we’d be doing that at the cost of other initiatives that could also be creating a million dollars a year at the 60, 70 kind of initial thresholds. And if you think about it, if we invest in addition to parallelizing the value in total, creating a lot more value faster in a system that is automating the learning process, these models are going to get from 60 to 70 to 80 with very little human intervention, as long as our users are actively using it.
So the more the system gets used, the more data flows through, the more the internal AI is going to be training new models, is going to find models that test more accurately than the models that are in production, surface those to a human for analysis who’s going to evaluate not only that model performance but also the cost benefit.
And that’s where the deep focus on the numbers both before the project starts as well as all the way through the project on an ongoing basis, are producing much, much better results and have for decades. I mean, this is just as true of traditional software that did this as it is of AI.
A lot more people got away without doing it with regular software. Virtually nobody’s getting away. There are very, very few exceptions, Scott, of people who are really creating meaningful value at scale on a consistent basis with AI, who are not doing this kind of deep economic analysis and really watching the spending thresholds, and balancing going deep into certain use cases versus going broader across others. You got to break it down this way to be able to maximize value for the organization.
Scott King:
Alright, we’ve discussed a lot about value in economics. It’s a good place to level-set everyone. Now, I’m kind of curious, and this might be a trick question, so apologies in advance. How important is it for organizations to have machine learning experts? We have customers that don’t have any machine learning people; they’re just using the software to do it. So, could you speak briefly on that, and then I have a wrap-up question for you.
Luther Birdzell:
Okay, Scott, I’ll respond as quickly as I can. However, I need to break down the market a little bit to answer this. It really depends whether we’re dealing with a big company, a medium-sized one, or a small one. So, talking about the Global 2000 companies, not only should every one of those companies have them, but also the Fortune 500 threshold in the US is about 4.5 billion. I think globally, in the 2000s, it’s somewhere in that $4 to $5 billion range. Companies of that size should have C-suite leadership either reporting directly to the CEO or CEO minus one, reporting into a CIO, CISO, CTO, or someone of that sort. They should absolutely have senior executive AI leadership that is responsible for the return on investment in AI technology. They should have some management level folks who have the experience, have done this successfully, and can mentor teams. Especially the bigger you get into that class, the large company class, the more teams they will want to be able to parallelize efforts. Those teams need to be coordinated with software engineers who have successfully deployed AI and ML. The “we were successful with software, therefore, we will be successful with AI the same way” approach is very flawed and often leads to failure. As the saying goes, the dumb repeat mistakes, the smart learn from them, the wise learn from others. There’s a wisdom opportunity here for people who have not yet wasted a lot of money trying to build AI the same way they build software. Then, there’s the middle market, Scott. A lot changes here. This is the part of the market I’m most excited about. My personal professional mission is to help teams succeed with AI, help humanity succeed with AI. I think we’d have the biggest impact in the middle market. For the 2,000 global companies, the biggest ones, there are about 200,000 to 250,000 companies in the United States alone with revenue between one million and one billion dollars. This is generally referred to as the middle market. Then there’s this huge number, roughly 33 million in the US alone, of small businesses that employ about half of America. This is my biggest concern. I don’t believe that the dry cleaner or ice cream parlor needs AI in the near term to continue to stay in business and employ a few of their neighbors, a few of the people in the community. If I’m wrong about that, life as we know it in the United States fundamentally changes. There would be a major, major socioeconomic change if small businesses can’t stay in business and half of the American workforce winds up having to do something else.
Scott King:
They’re all swallowed by Amazon, right?
Luther Birdzell:
Yes, there’s going to be a bit of that. However, I believe many local businesses, such as the ones I frequently patron, will continue to exist for the foreseeable future. I can’t predict what’s going to happen 10 to 20 years from now, but I believe many of these businesses will weather the AI cycle just fine.
The middle market faces a challenging landscape where many businesses will not survive if they fail to succeed with AI. Some companies, if they do succeed with AI, will expand and dominate market segments, possibly even opening up new markets to become key players. However, these companies don’t have the balance sheets to force AI implementation like the big players do.
Even within the Global 2000 companies, there’s a vast difference between the bottom $4 or $5 billion companies and multiple hundreds of billions of dollars of companies at the top, such as Shell and a few energy companies. This disparity presents an array of options for the larger companies which smaller companies lack.
For medium-sized companies to survive, they must succeed with AI, or they will disappear. They have to execute this at a much lower cost than anyone has managed to do so far. To achieve this, they must heavily rely on automation, much more than ever before.
Automating the learning process allows these companies to undertake multiple projects with a single data science team. Lower middle market companies might not have the balance sheets or capital to staff dedicated teams, but middle and upper-middle market companies do. The companies that will truly succeed are those able to staff one or two teams that can handle five to ten projects.
This is achievable only by automating the entire process, including connecting the AI with the business. If you add intelligent automation software, it can track what’s going in and what’s going out, even interfacing with humans when necessary. Not only does this provide excellent audit records for your GRC requirements, but the same data can be used to continuously train the machine learning and AI currently in production.
This allows AI to lead the ongoing optimization of AI by making suggestions to the data science team to evaluate whether the new model being suggested is better than what’s in production. If it’s not, they move on. If it is, they might start a short approval process to automate the deployment into production. This changes the cost basis and the economics completely.
As the company gains momentum by creating high ROI with their AI projects, the cost savings and value creation from these projects can fund other projects. This allows them to grow AI teams and data science teams using the value they’ve created with the technology already. However, you can’t reach this point unless you have the automation to keep the cost of ownership low enough to actually capture those returns on investment.
Scott King:
Super. Those were some great sound bites. I’m definitely going to use some of those in the future. I appreciate your time, Luther. And thanks to everyone for joining us.
Luther Birdzell:
Hey, Scott, thank you so much. Let’s do it again soon.
Scott King:
Alright, we will. Thanks, everyone.
Read our comprehensive LLM testing guide
See how several LLMs fared in our test and steps to run your own tests for each of your use cases.
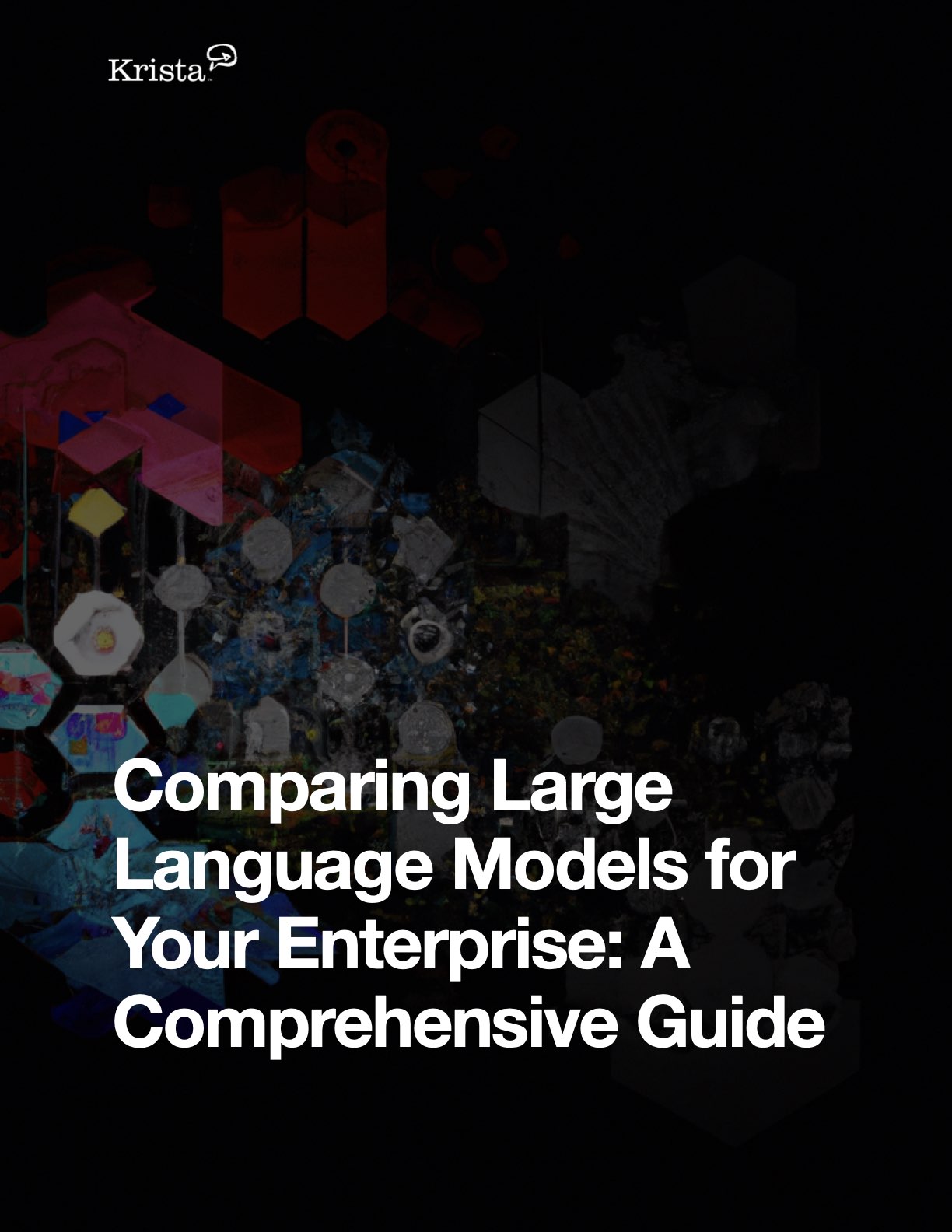