2024 AI Predictions
What does the internet say about AI?
What do the AI pundits think will happen?
We were curious, too.
In our quest to understand what was being predicted for AI in 2024, we reviewed a set of diverse sources to analyze and merge a myriad of predictions to provide a consolidated overview. This article cuts through the noise, delivering a straightforward perspective on AI trends. We’ve factored in common predictions and outliers, providing you with a balanced view of who predicts what when it comes to AI.
The Sources Behind AI Predictions
In our review of AI predictions, each source offered distinct insights reflecting their unique perspectives. I’ve linked each of the sources from Adobe, Forrester, Gartner, IBM, IDC, LA Times, Nvidia, PWC, TechCrunch, and TechTarget that we reviewed and categorized.
- IBM emphasized predictions at an enterprise level, focusing on how AI would reshape business operations and strategies.
- Gartner and Forrester focused on the impact of AI on individual task levels, highlighting how AI could enhance personal efficiency and workplace dynamics.
- IDC provided a more IT-centric view, exploring how AI would aid IT professionals in their roles, with an emphasis on shifting outcomes and the emergence of conversations as the standard user interface.
- LA Times, PWC, and TechTarget brought attention to the coming of age of open-source AI, stressing the importance of ethical AI and the need for transparency in AI operations.
- Nvidia presented a broader spectrum of insights, reflecting the diversity of opinions from the 17 experts they consulted, covering a wide range of AI applications and implications across various sectors and disciplines.
The AI Landscape – A Consensus View
Across the board, experts agree that generative AI is set to skyrocket this year, bolstering productivity and spurring innovation. Businesses are bound to see a significant shift towards multimodal AI, which invites a more natural interaction with technology using voice, images, and text. As these technologies advance, tight AI regulation is expected to emerge, guiding their integration into the market. The consensus is clear — AI is not just a fleeting trend but an innovation that is fueling economic growth and investments.
Consistent Predictions
- Generative AI Expansion: Broad consensus on the significant growth of GenAI applications across industries.
- Multimodal AI Advancements: Widespread agreement on AI’s evolution to include various input modes (text, voice, image).
- Regulatory Landscape: Uniform anticipation of increased AI regulations with varying regional approaches.
- Economic Significance: Collective prediction of AI’s growing impact on economic indicators and continued investment surge.
Outliers – Unique Predictions and Their Significance
Not all forecasts follow a common thread. Gartner casts a spotlight on AI’s role as an emerging economic indicator of national power by 2027. Meanwhile, TechCrunch raises concerns about AI’s potential misuse in the 2024 elections. Nvidia equates the race for AI supremacy to a new space race. These outlier predictions, while not widely echoed, provide insights for businesses to consider, presenting both opportunities and warnings.
Notable Outliers
- National Power Indicator (Gartner): AI productivity as a measure of national power.
- Unionization Surge (Gartner): Predicted increase in unionization due to GenAI.
- Election Disinformation Concern (TechCrunch): AI’s potential negative role in the 2024 election.
- AI Risk Insurance (Forrester): Introduction of AI hallucination risk insurance policies.
- AI ‘Space Race’ (Nvidia): Nations developing AI capabilities akin to a space race.
- Robotics Industrial Impact (Nvidia): Significant use of AI and robotics in healthcare and manufacturing.
Implications for Business Deployment of AI
For business leaders, the consensus and outliers translate to a clear message: adapt and adopt. Integrating AI into your business requires a balance of strategic foresight and practical application. You have to stay agile, informed, and ready to pivot as AI technologies and regulations evolve.
To stay ahead, businesses must embrace AI, weaving it into the fabric of their strategies. This means not only leveraging AI’s capabilities but also navigating its complexities with a keen eye on governance and ethics. The key lies in a proactive approach to adopting AI — one that aligns with improving business outcomes and making things simpler for your people and your customers.
Start Deploying AI Now
AI undoubtedly provides a competitive edge, so don’t just observe what is happening. Act now! Your proactive engagement with AI will keep you on pace with those who have already started reengineering how work is done. Your use of AI will not be unique, but your journey will be. Begin your AI journey today. Reach out to experts, participate in workshops, and integrate AI into simple workflows to achieve quick wins and fast ROI. We have lots of example use cases to choose from, so don’t be an observer. Step forward and contact us so we can share insights for your unique journey.
Links and Resources
- Top 6 predictions for AI advancements and trends in 2024 by IBM
- What AI will bring in 2024: 4 predictions by The LA Times
- 2024 AI Business Predictions: PwC
- AI trends 2024: Predictions for the next wave of business innovation by Adobe
- 8 predictions for AI in 2024 by TechCrunch
- 17 Predictions for 2024: From RAG to Riches to Beatlemania and National Treasures by NVIDIA
- Gartner’s Top Strategic Predictions for 2024 and Beyond
- Predictions 2024: Generative AI Transitions From Hype To Intent by Forrester
- 6 generative AI predictions for 2024 by TechTarget
- Top 10 Worldwide Artificial Intelligence and Automation 2024 Predictions by IDC
Speakers
Transcription
Scott King: Hey everyone, I’m Scott King, and that is Chris Kraus. Hi Chris.
Chris Kraus: Hey, how’s it going, Scott?
Scott King: It’s going well, and thanks for joining this latest episode of the Union Podcast. We’re going to talk about the AI outlook for this year, this year being 2024. So Chris and I scraped the internet, and we picked 10 different articles that predicted different outcomes and different theories and hypotheses of what AI is going to do in 2024. So we want to take you guys through that, let you know what we found, and ask for your feedback. So Chris, any opening remarks before we get started?
Chris Kraus: I thought it was interesting because of the sources you found for some of our software companies; some of them were news media, and some people were consultancies. So it is, they’re diverse in what they, their backgrounds. I think it was interesting because their predictions did kind of follow in line with what they do, right? And their core business itself. I would say they’re always skewed based on what their subject matter expertise is.
Scott King: Yeah, they definitely write for a specific audience. And I purposely didn’t pick just enterprise publications because it would just skew the results, and everyone is worried about AI bias anyway. So I tried to remove that. So we picked sources from IBM, the LA Times, PwC, Adobe, TechCrunch, NVIDIA, Gartner, Forrester, TechTarget, and IDC.
And of course, I’ll give you guys all the links that we read, all the articles that we read. But what we want to do is just give a high-level overview of what we found and then take you through some of the technical categories and AI categories and how they ranked at one, level of impact, and two, how many people agreed or disagreed with that. And there are some really interesting outliers that we want to finish with on the predictions. But first, Chris, let’s talk about IBM. IBM is a partner company of ours, and they have a really good prediction article. What was the flavor of the IBM article, and what do you think they were trying to say?
Chris Kraus: Well, what I thought was interesting is they’re very enterprise-focused. They talked about deploying open-source and microservices. So their stuff was less about an individual tasking at the desktop, but actually, okay, if you make this a service, how can you apply it across the business and the enterprise? So I thought that was interesting. They were very enterprise-focused. Look at the big picture. Don’t look at a single step, a single process itself.
Scott King: Yeah, and I mean, that makes sense, right? That’s their audience, right? Enterprise businesses and SMB businesses are trying to install all types of different software, hardware, and applications. I can’t imagine what the IBM SKU list is, but it’s gotta be big. So everyone talks to Forrester and Gartner. They want to know what Forrester and Gartner say because they must know everything about everything. I read Gartner and Forrester, and they definitely gave us a little bit. A lot of the content was behind, like a paywall, or they did a webinar, and you had to listen to that. So we scraped basically the preamble to their big content piece. What did you find interesting in there?
Scott King: Well, they were definitely focused on the individual and like a personal knowledge worker. So, on individual tasks, talking about individuals in a company using AI to accomplish things at their desktops or what they’re doing, they looked at whether there will be a difference between low-cost countries versus higher-cost countries. How much traction are you gonna get? Because a lot of times the saving at a task level is based on your hourly rate. And so their predictions always aligned with knowledge workers, what people are doing at the desktop and that. So very focused on things that are tangible that people would see.
Scott King: Yeah, I find that interesting because I would want them more similar to IBM than they were. And then to juxtapose that, we chose IDC. So IDC’s article was a little bit longer, and it’s a little bit more of what I would expect an analyst to say about AI predictions. They talked about shifting from what you were talking about, the task level orientation, how AI can help me write an article, create an image, and things like that. They’re really talking about shifting to outcomes. Think of all of the pieces strung together as a business outcome. And they said that was important, which obviously I like because that’s what we do.
Chris Kraus: Yeah, well, the other thing is they talked about modernization. So a lot of their stuff was how do you use this to help things that IT would do like testing, application development, application modernization. I mean, we’ve seen a lot of good examples of people talking about reading old COBOL programs with AI and generating modern Java code that does the same thing because people forgot what those business rules do from the 80s and 90s, probably still some from the 70s. So I think their focus is very tangible, especially for IT operations, to make yourself more efficient. And the outcome is if you can modernize into modern code, then anybody can read it. More people know Java and how to maintain that than legacy cobalt or assembler, RPG, and all those cool things like that.
Scott King: You know, that is, yeah, that’s interesting. So my father-in-law was a COBOL programmer at Chase Bank, right? And he always told them he was going to retire, and they would just keep paying him more and more money, right? Because like, Hey, nobody knows how to work on this stuff anymore. We need you to hang around. So if anyone was worried about AI taking their job, it’s that one. And that’s a good reason because those guys aren’t in the workforce anymore. So we need to modernize all those, modernize those applications.
Chris Kraus: Great. Thanks for pointing out that I have gray hair and my first job out in college is writing COBOL for batch jobs and online systems, Scott.
Scott King: Yeah, well, and we can’t get out of the testing business. Like I really appreciated that they were talking about software quality. And so that’s always big, right? If something doesn’t work, it totally blows your mind because you think the internet should work all the time; every application should work all the time because the consumer applications, Facebook, Instagram, Twitter, well not so much Twitter, that breaks quite often now.
But, you know, they spend so much time on software quality that that’s extra expectations. So if you go to your bank, as I went to, I think, Forbes yesterday. Forbes was down. And that is why they’re not in our analysis, right? Because I was like, OK, I want to read this article from Forbes. The website didn’t even work. So I was like, OK, moving on.
Chris Kraus: That’s what we do these days, right?
Scott King: All right. Yeah, the other, so we did pick some, you know, more, I would say, I mean, still business oriented, it’s still technical oriented, but more consumer-oriented articles from LA Times, Tech Target, and Adobe because those seem more like task oriented like we were talking about. What did you find out about those articles that were interesting?
Chris Kraus: So I thought it was interesting. And I think Pricewaterhouse, PWC, fell into this category also. They were really focusing on, okay, it’s coming of age, but how do we deal with the ethics? And just, you know, it’s something we’ve talked about. Will there be government regulation on ethics? Will we get guidance? How do you get transparency in what the AI models are doing? So they were talking about it being actually applied and used, but not about how it’s gonna be used, but actually, like ethically, how are we gonna use it? What’s the transparency we have to have? And so I thought that was refreshing to say, it was kind of a given for them, people are gonna do this. Let’s talk about management, let’s talk about the ethics and the higher level of the use of the technology itself. So they were kind of, it was a given, you’re gonna go do this, how do we manage it in an ethical way? And so they weren’t ever talking about preventing it and stopping it.
It’s so I thought that was refreshing to see. They’re kind of it’s a given. Of course, you’re going to do this.
Scott King: Yeah. Of course, you’re going to do this, but just where are you going to apply it? And that’s why we kind of created these categories. So we took all of the predictions and put them in four different categories, the economic impact, the regulatory changes, risk management, and basically just advancements in the technology. And then we measured that on the level of impact in our opinion, right? But the first one was economic impact; Chris and most of the articles agree. There’s a huge economic impact, there’s a huge lift, everyone is gonna do this, they just have to figure out how. And then there was one outlier in this economic impact that I thought was super interesting, but it wasn’t a prediction for 2024. They put it all the way in 2027. Gartner said, okay, the national power indicator of the country and the GDP is how good you do AI. Any thoughts on that?
Chris Kraus: Yep. Well, it was interesting to put it in 2027. That gives us the leeway to, say, solve actual power problems, to have enough CPUs and cooling power to do it, to actually figure out how we’re going to handle the ethics and actually put it in production long enough. So I think that means that from 24, 25, 26, people are going to be time on task implementing this so that in 27 there will actually be an indicator. So I like the fact that they were kind of long term and saying this isn’t a flash in the pan, you know, none of the troth of disillusionment and that kind of stuff actually giving us a good long term look.
Scott King: Yes, I appreciate that. Most of their predictions, if you read them year over year, they will do this year, and then they’ll throw some stuff way out three, four, five years out. And then they comment on that, like, oh, we predicted this, and it didn’t really happen.
Chris Kraus: Yeah, well, so does the stem sentence, but we do comment on that.
Scott King: Then the next was regulatory changes. And most everyone said there’s going to be advancements in AI regulations. You have to look at this in terms of what is helping you do, how you measure those outcomes, and then basically how you version your models and bias and all that. So I thought that completely made sense. There weren’t really any outliers in there. So, we didn’t label any outliers because almost everyone agreed. But the high level of impact, right? Because you can only do what you can do. And then risk management. I thought this was super interesting because there was way more content about risk management than I expected when I started. So I’m glad we categorized all these predictions with risk management. Obviously, the predictions will, they stated that. We’re going to have to have advancements for how to measure risk, get more data, mitigate that risk, and understand your business. But there are a couple of outliers.
What were the outliers, Chris?
Do you have the chart in front of you?
Chris Kraus: So, yeah, one of them was actually AI risk insurance. And Forrester talked about that. So would we have something like AI insurance the same way we have cyber insurance, health insurance, or liability insurance? So will people solve the risk by actually applying an insurance policy to it? That’s GRC 101. If you’ve got a risk, how do you mitigate it?
And then the other was the unionization. So this was really interesting to me because it would have never dawned on me that IT workers would actually join a union. We hear about, like on the news lately, there’s the Pilots Union and the Fire and Police Union, there’s UPS and the auto workers, and those people actually doing things, they’re very hands-on tactical, they’re providing services.
They’re building cars in that, but this was talking about whether will we have unionization for people with intellectual property knowledge and knowledge, and like you mentioned, your Father in law and being a COBOL programmer could be replaced by AI will we see unionization in the white collar workforce around knowledge work and that’s kind of weird to me, but because I would think that most of the things are things I don’t do well anyway, like please correct my grammar and my spelling, right? So, um, so I was kind of torn on that, but they said, you know, that is a potential there.
Scott King: Yeah, yeah. And then it is interesting that it was from Gartner as well. So they had more outliers than anybody else. So I don’t know what that says. But obviously, since the generative AI boom and everyone is super interested in all these different AI products, the advancements in the technology. Of course, everyone agreed, and they said, okay, it has a high level of impact, right? The most level of impact because we’re going to change the way we serve the customers, right? And employees, external customers, and internal customers. But the big was the multimodal functions of AI and then the integration, right? Because if you have, we’ll go back to that task level comment that we had at the beginning. So if you have tasks that are being supplemented with AI, but that’s inside of a larger process, obviously, you have to integrate those. And then the multimodal, yeah, I mean, imagine with the images and cameras and voice and text, and that’s super, super interesting.
Chris Kraus: Yep. Well, so we know that say, in a warehouse, just like in an Amazon warehouse, so they’re kind of famous for this. You put like the seven types of Barbie dolls on different shelves, so you don’t accidentally pick the one next to it, you know, picking the wrong model of things and that. So they purposely make their warehouse shelves random, so it’s easier for a human to identify, oh, this is what I’m picking, because it’s definitely not the things on either side. And we have that in, like my doorbell camera, it doesn’t matter if it’s a Home kind, it’s a Ring, it’s an Arlo, they’ve had package detection for years, right? So I get a notification you have a package versus, you know, someone’s at the door or who’s at the door, things like that. So I think that those types of things, and Nvidia talked about robotics, are going to come together, right? So we’re going to see not crazy, crazy things like I’m going to walk into the gap, and they’re going to identify my size and tell me what to buy. It’s going to be very practical. Like if we say we’re going to get better with AI and robotics, doing, is it getting, does it know which, what the difference between a breaker box and a light panel and how to flip something or things like that. I guess that’s what Nvidia called the space race because there are a lot of things where AI can enhance robots. So like, when it’s welding a car, could it pre-identify the frame of the car and the model of the car versus being told? So there’s probably a lot of very practical things there that we’re not even thinking about.
Scott King: Back in the seventies and eighties, you didn’t want to buy a car that was built on a Monday or a Friday. Because either the unionized crew, so here we are in the union again, the unionized crew is either tired on Monday or they’re looking forward to the weekend on Friday. So those cars just weren’t built well, like AI would solve that problem. But yeah, the Nvidia, of course, I mean, they’re making the chips. There’s a huge demand for that. And, of course, their view was very hardware and then embedded software related. I did, you know, you’re talking about the robot. I got a demo from the IBM Innovation Lab here in town. They had one of those, the yellow dogs; what is it, Boston Dynamics, is that what it is? But they had programmed one of these dogs to walk around a warehouse, not an Amazon; it could be an Amazon warehouse, like you mentioned, but they were looking at the pressure gauge and the quality gauge on fire extinguishers. So instead of a person running around, they just said, okay, dog. I need the needle to be in the green. Here’s a map of all the fire extinguishers. The dog never gets tired. The dog just goes and collects the data. So I thought that was super interesting. So, of course, that is multimodal. Have it take the image, reading the offline device, right? If you had an internet connected, you wouldn’t need that. But a fire extinguisher, you don’t really want it plugged into the wall. That’s called a fire hose.
Chris Kraus: Right. And that doesn’t seem scary to me. Like, that’s very practical. Like, hey, robotic dog, look at all the fire extinguishers. Check the gauges. Let me know if any of them need to be recharged or replaced. That doesn’t seem scary to me at all. That seems very practical. So, you know, in every movie, the robot’s gonna come smarter and kill people. But in that case, it’s actually something that doesn’t bother me at all, right? I think another way you could see that is the local gas company, when they inspect the meters actually people walk around with the sniffing machines, house to house to house to house, and they say the crew, they say they move from town to town across Texas.
And so they can only do it like every other year. But I was like, one of those robotic dogs could actually hold the sniffer, walk up to every gas meter, test it, and go to the next one really quick. So would that take away jobs from people? Or just let them focus on, OK, these are the ones that flagged; double check the ones that have a problem. There’s probably a lot of really cool things in robotics. Say this is an electric meter, this is a gas meter, things like that.
Scott King: Yeah, they still read by the water meter in the street. A person walks by, takes the lid off, and scans it with some type of optical device. You can’t do that for long, walking around and bending over every day a thousand times. Yeah, you should have a robot do that. But the old infrastructure, like newer buildings, right? They can read it in one spot, but in the older neighborhoods, all that is disconnected. All right, so we went through what we learned from all the different sources; we talked about the different categories, who agreed, and who had outliers. Any closing thoughts on what people should be listening to us, hopefully they’re still listening, and what they should do in terms of looking at AI, either from a task level, from an outcome level, or from a conversation level?
What’s your recommendation?
Chris Kraus: Well, everybody agreed you need to do something. It’s, this is, we’re starting to operationalize this, whether it’s at the task level or the enterprise level. So, this is no longer the science experiments we’re talking about. We’re talking about deploying and getting things done. So, in 2027, we’ll have a major economic impact. So I thought that was interesting. And like Scott, remember we talked to a group of, at an investment firm, and they’re like, you know, a robot’s gonna take over the world and kill us. It’s like, no, no one’s actually predicting that.
They’re actually predicting AI with robots doing helpful things. And so we’re, this isn’t going to be big brother looking at us and those type things. Everything, no one had big fears. They were very, let’s, we’re gonna do this, we need to put ethics behind it, but it’s gonna help people do tasks, it’s gonna help you come to an outcome in the enterprise, it’s gonna help you rewrite, modernize applications. I thought everything was very positive, so we don’t have to worry about, you know, Skynet proverbially coming and getting us.
Scott King: Yeah, I think, yeah, I do remember the meeting at the investment firm. They did talk about robots, and half of us giggled about it. But what I thought was really interesting is they took the next level, and they thought about the, the unintended consequences because they said, we’re all going to do this and then, okay, what happens next year or two years down the line and three years down the line? Like, like our business supply chain, especially a talent supply chain, is gonna so significantly change we don’t understand how to work that. So that was super interesting. That’s why no one, like no one in these articles, talked about talent and skills.
Chris Kraus: Yeah, no, the change of reskilling, how do you reskill people? You would have thought that would have been a prediction, that there would be a market for reskilling people, but they really didn’t talk about that, now that you say it.
Scott King: Yeah. So yeah, if you’re listening and you want to be into that, I think someone should start a business. Or you can go on the internet and find all the AI prompt gurus. You can buy their class for $25. Well, I appreciate it, Chris. Thanks. This was a fun project. We actually used AI to look at all the different categories, scan all the articles, and come up with some summarizations. So that was a super helpful exercise, and I really appreciate your outlook on this and your advice to all of our listeners.
Chris Kraus: Thanks, Scott.
Scott King: All right, until next time, bye.
Read our comprehensive LLM testing guide
See how several LLMs fared in our test and steps to run your own tests for each of your use cases.
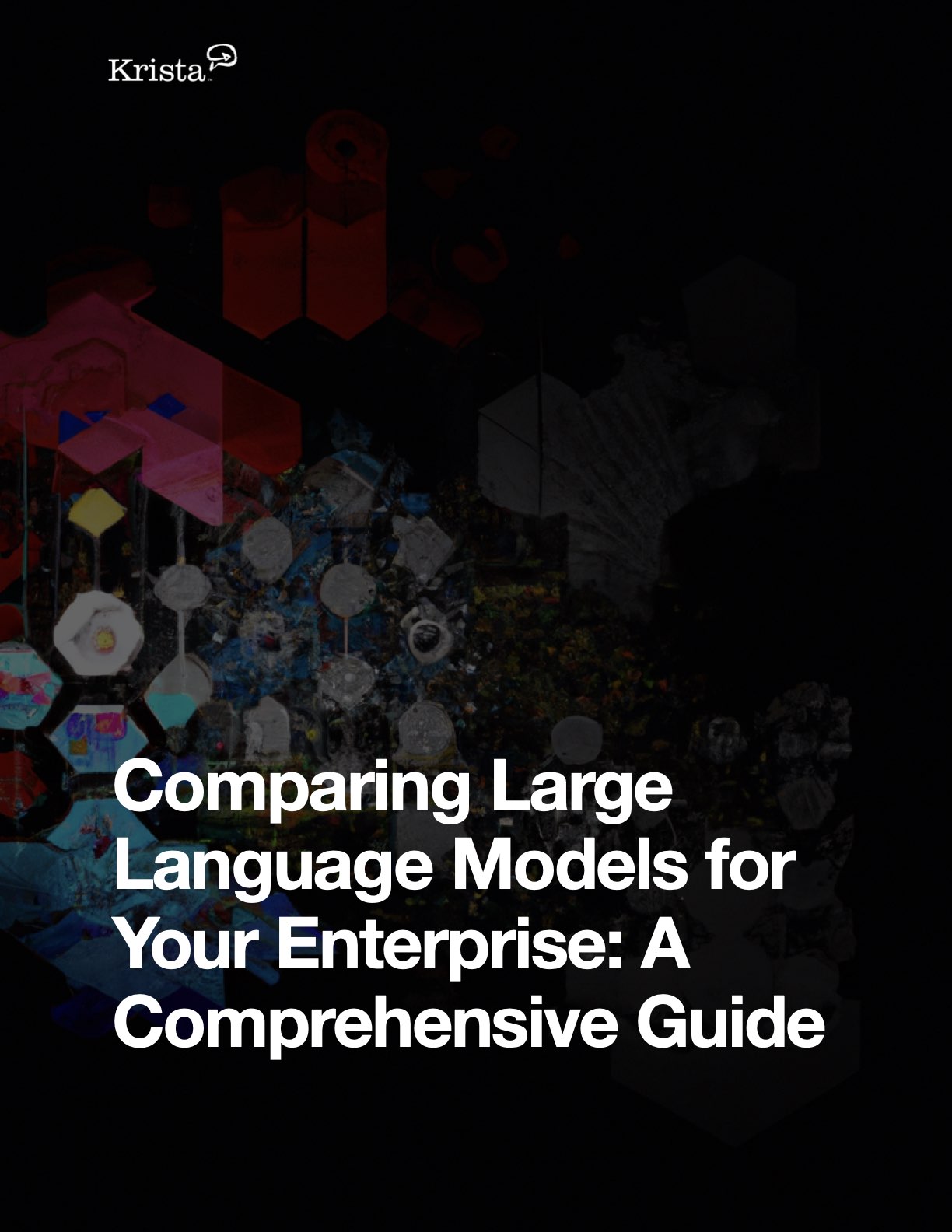