Artificial intelligence (AI) has an immeasurable impact on various industries, from finance to healthcare to customer service. It can automate repetitive tasks, derive insights from massive data sets, and even help manage and govern data. However, effectively governing AI data requires a well-thought-out strategy and proper implementation. Chris Kraus and I discussed the importance of data governance in AI and how to effectively manage it in this episode of The Union.
AI Should Involve People in the Same Context
Any AI conversation should maintain a ‘shared context’. A shared context refers to the ability of an AI system to maintain a consistent understanding of a situation across multiple interactions and even multiple users. For example, in a customer service scenario, a shared context would allow a customer service agent to pick up where a previous interaction left off, saving the customer from having to repeat information.
Maintaining a shared context across multiple interactions is crucial since customers may require help from multiple systems or people spanning several sessions. Therefore, AI systems should be able to manage long-running conversations and provide previous knowledge. Many conversations are not resolved on the first attempt so any AI system should be able to recognize that it is the same conversation and maintain the same context.
AI Should Know Who Is Allowed to Know What!
When implementing generative AI for customer or employee assistants, it is critical to understand who has access to what data and for what reasons. With many different stakeholders involved, from employees to managers to end users, you cannot afford to expose data to those who should not have access to it.
For instance, a call center agent may need to consult with a manager to approve a customer interaction or an offer. This decision may involve accessing customer data that the agent does not have the privilege to view. An automated AI workflow can handle this situation by learning how such decisions have been made in the past and using that knowledge to decide on its own, without the need for human intervention. However, it’s essential to ensure that AI does not provide answers to those who should not have them, maintaining data privacy and security. You wouldn’t want employees to ask the HR or payroll systems questions about employee health, benefits, or salary information.
AI Conversations Should Transfer from Chat in a Browser to Mobile Platforms to SMS
Today’s customers expect a seamless experience across all platforms, whether it’s desktop, mobile, or SMS. By enabling ‘long-running conversations’ across these platforms, businesses can enhance omnichannel customer experiences while also governing the data that supports them.
For instance, customers may start a conversation via a chat on a desktop, then move to a mobile app, and finally to SMS. This seamless experience across the omnichannel is only possible if your AI can manage data effectively across these platforms.
You should invest in technology capable of supporting multi-channel interactions and maintaining long-running conversations across these channels. Many stakeholders often get stuck thinking in terms of screens and forms like legacy applications rather than conversations. This mindset shift is necessary to take full advantage of AI’s potential to manage and govern data effectively.
Effectively governing AI data involves maintaining a shared context across many people, understanding who is allowed to access what data, and managing data across multiple user interfaces. By focusing on these areas, you can reap the benefits of AI while also ensuring your data is managed effectively and securely.
Links and Resources
Speakers
Transcription
Scott King:
Thanks for joining me, Chris. This is the third episode of our three-part series on integrating ChatGPT, Bard, Watsonx, or any other generative AI into your business. We’ve had a great journey so far. In the first episode, we discussed the integration of generative AI into the enterprise, while the second episode focused on how AI can assist us in an automated manner.
In this episode, we’re exploring governance—controlling access and managing data. It’s essential not to give everyone access to everything. We need to practice the principle of least privilege and ensure that different roles have access according to their responsibilities. Furthermore, we need to discuss how to maintain consistent context across various channels, such as chat, mobile app, and browser, and how to provide everyone with the required knowledge for automation. Let’s delve into a specific example, say, customer service.
Chris Kraus:
Indeed. As we approach our last episode, it’s important to note that we’ll need a new example next week. But for now, let’s focus on customer service.
In customer service, you typically have two key roles—the consumer and the contact center agent or AI responding to queries. Our goal is to move beyond simple FAQ lookups and towards accomplishing tasks.
When a customer service agent receives an escalated request, they should have access to the customer’s history, as well as a comprehensive view of the customer—open tickets, pending returns, invoices, and so on. We want to provide them with read access to this information, helping them understand the customer’s situation better.
Furthermore, in situations where a request needs to be escalated to a manager for approval or order override, the manager may have elevated privileges. For instance, an agent might only be able to view orders but not update them.
This context is crucial in automation. It involves two aspects—workflow rules and role-based access control and the availability of AI commands that aren’t accessible to everyone.
Old-fashioned apps used to handle these tasks, but now we want AI to bring these elements together in the context of a conversation. It simplifies the workflow and enhances the customer’s experience.
The principle of least privilege you mentioned, Scott, is critical. We need to secure our data accordingly. For instance, when storing data, we must include validity dates to ensure the correct policies are applied. Additionally, we must decide how much detail an agent can access. For example, should an agent see the lifetime of orders or only the last few open ones?
Another instance would be HR documents and manuals. Inside the company, managers may see pay grades and bonus structures, but employees can’t. This information should be secured based on role-based access control. The term most people use for this is ‘least privilege.’ As we’ve experienced in a large company, you know the salary bands exist but not the details behind them.
Scott King:
Indeed. A manager would have more detailed knowledge. I also thought about the call center agent scenario. We’ve all had instances where we’re seeking help, and the call center agent puts us on hold while they access another system or escalate the issue. In an automated workflow, AI should learn from these processes and potentially make decisions based on historical data.
Chris Kraus:
Precisely. The models can learn categorizations and predictions. Generative AI can provide a sensible response, but machine learning also provides categorizations. For instance, deciding on a discount—yes or no, or maybe 10% or 20%. If you escalate to a manager, they review the customer profile and make a decision based on their history, value, on-time payments, etc. Machine learning can be trained on these examples, and over time, it can start suggesting actions. At some point, the AI can take over this decision-making process, especially for repetitive questions. There are also confidence metrics that can help decide when to escalate to a manager. It’s certainly more efficient than leaving a customer on hold while an agent consults different systems.
Scott King:
That’s true. It’s often a combination of consulting other people and different systems. Companies that have grown through acquisitions often maintain multiple ERP and CRM systems, complicating the job of the contact center employee, who often has the least training but the most complex task. Understanding data governance and who is allowed to do what can be quite complex in a large organization.
Chris Kraus:
Absolutely. When this knowledge is built into the model, it can better determine whether it can provide an answer or access certain data. Anyone can ask any question; we just need to ensure we’re not providing answers to those who shouldn’t have them. The AI could either say it can’t provide an answer or give more details about the information. Trust in AI will increase when people realize it won’t answer every question or fabricate answers.
Scott King:
That’s a good point. There are stories of generative AI making things up based on language patterns. So governance is crucial to ensure it doesn’t provide inappropriate or incorrect responses. When uncertain, the AI should indicate that it doesn’t know the answer and might need to elevate the conversation to a phone call. As we try to govern this data across multiple interfaces—chat windows, mobile, text messaging, even WhatsApp—how does it all work?
Chris Kraus:
Correct. The technology you use needs to be able to support multiple channels and maintain long-running conversations across these channels. For example, if a customer accesses your service through their mobile app, they might be identified by their phone number. If they switch to a browser, they might have to identify themselves with an email or the same phone number to maintain the continuity of the session. You want to avoid customers having to start over with their data repeatedly. This continuity should also extend to the call center agent. If a customer has been interacting with a chatbot, the agent should have a history of those interactions instead of re-asking the same questions. The idea is to have a shared context across different communication platforms, even if it means transferring from a digital channel to a phone conversation with a human agent. This continuity enhances customer service by maintaining a long-running conversation across different channels. Whether the customer starts on a browser, switches to their phone, and later returns to their computer, the experience should be seamless. This can be achieved with generative AI or different AI models that allow customers to continue asking questions until they get their desired results. This process should reduce contact time by eliminating the need for customers to repeatedly explain their problem to different agents.
Scott King:
That’s fascinating. You mentioned long-running conversations and sessions multiple times. Could you quantify that? How long is long and how many times can a customer go in and out while maintaining a single session?
Chris Kraus:
This could be user-configurable. However, a session could last for two weeks, for instance. If a customer is trying to resolve a complex issue, they might need this extended period. But in most cases, a few hours to a day should be sufficient. If we consider an employee onboarding process, it might take up to two weeks, as they would need to complete training classes, receive their hardware, and so on. But for assisting a call center, one or two days should suffice to retain the history and conclude the matter. That doesn’t mean we discard this information afterwards.
Scott King:
Interesting. It’s like having multiple people inside the same context. Even when someone leaves and returns to the conversation, they can still remember what they were discussing.
Chris Kraus:
Exactly, we need to continue conversations across channels and over several days to improve the user or customer experience. Everyone dislikes having to repeat themselves and start over, so the goal is to continue where the conversation left off.
Scott King:
So, it’s possible to have automation that supports all our data governance and compliance policies. It understands where our data is and who has access to it, supports long-running conversations, and considers multiple channels. I think of situations like customer support and service, in the telecom or TV industry, for example. We’ve all had interactions like these. But while all this makes sense, some people might think it’s too difficult to implement. With something like Krista, though, that’s what we aim to do. Where do you think people would hesitate or think this isn’t possible?
Chris Kraus:
I believe people are accustomed to classic screens and forms rather than conversations. However, written conversation can work in WhatsApp, a mobile app, a browser, a chat window, etc. because we’ve simplified interaction to natural language. This means we can be available on every channel. I think traditionally, you’d question whether a mobile app looks like the webpage app and what to do if it’s a message. But by using natural language processing to communicate with users across all channels, we’ve lowered the technical bar. The first step is realizing we can have a conversation on any channel. As for software understanding sessions and users, that’s all technical stuff that developers handle for you.
Scott King:
That makes sense. It goes back to our previous episodes where we discussed using humans as the integration method. Our conversation in Teams and iMessage is the same conversation happening in two channels, but it’s only integrated in our brains. We need to shift this type of process to a platform, so when a new employee or a new customer faces the same situation, we can handle it efficiently.
Chris Kraus:
Yes, exactly. That’s the goal.
Scott King:
That makes sense. I’ll find that episode and link it. Thanks to everyone for joining us to discuss data governance, compliance, and multi-omnichannel conversations. Be sure to catch our previous episodes on how to integrate this into your enterprise. And then the second one, Chris, was about actually doing things, right?
Chris Kraus:
Yes, exactly.
Scott King:
So, it’s about how to get AI and automation to provide guidance, right? The next best actions and actually letting you complete a business outcome. So, this is the last of this series. I’ve enjoyed talking with you, Chris, about this. Until next time.
Chris Kraus:
Cheers.
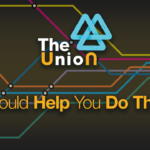
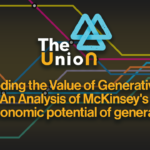
Read our comprehensive LLM testing guide
See how several LLMs fared in our test and steps to run your own tests for each of your use cases.
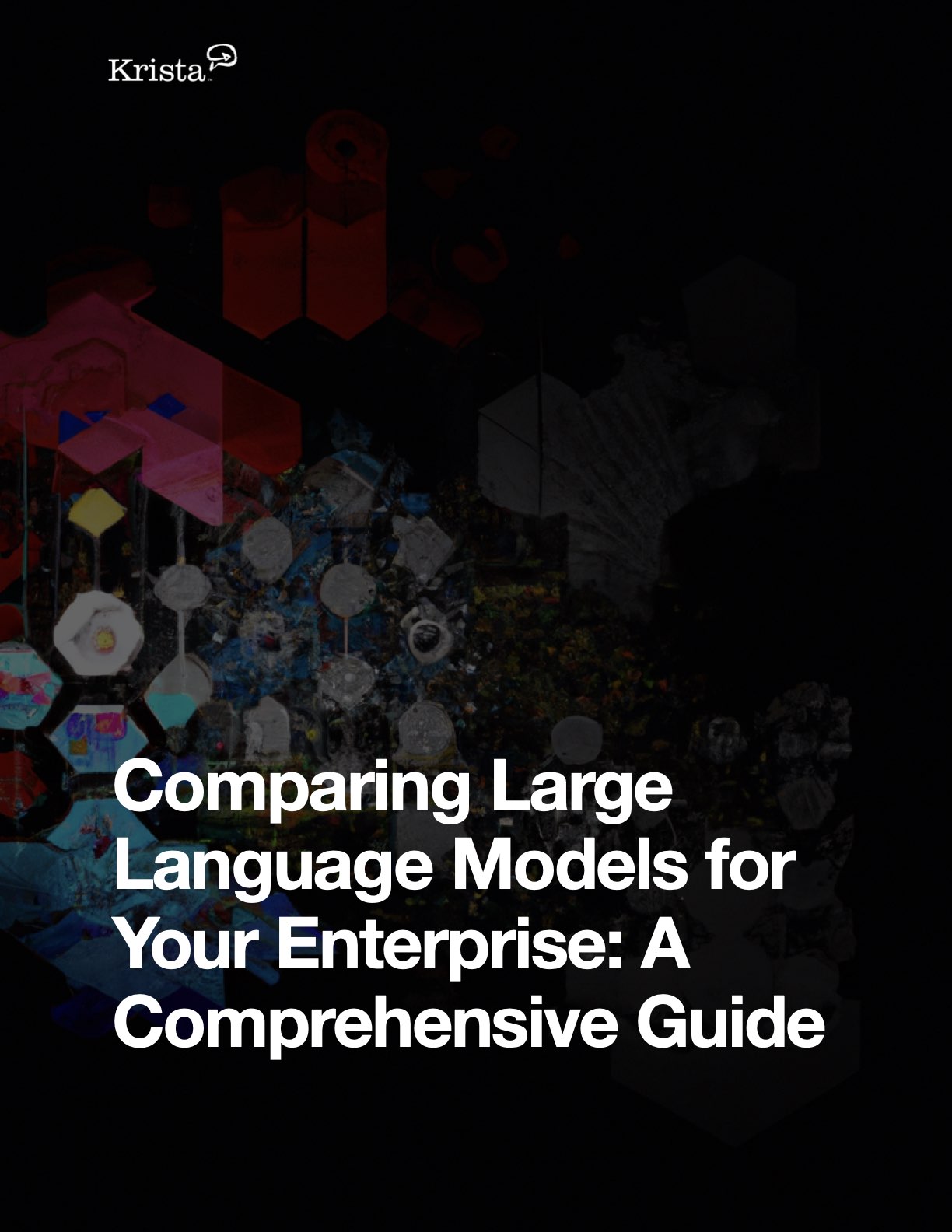