Generative AI is transforming how data and content are generated in many types of use cases. Its ability to quickly summarize data and enable people to interact with systems in a conversational manner is redefining what work is and the value associated with it. In a recent conversation with Chris Kraus, we reviewed McKinsey & Co.’s, “The economic potential of generative AI: The next productivity frontier” on how generative AI is shaping different roles across several industries, particularly in customer service, labor cost efficiency, and the implications for high-value work.
Generative AI assists in front and back office tasks
The line between back office and front office operations is blurring as generative AI takes on a more significant role. AI’s ability to assist in automating tasks previously managed by humans in areas such as customer service is remarkable. For example, consider the scenario where AI meticulously examines incoming documents in a loan application process, distinguishes them, and then forwards them to a loan officer for approval. AI aids professionals by automating routine tasks, allowing them to focus on higher-priority matters.
Generative AI ROI
One cannot overlook the financial implications of AI. In certain regions where labor costs are low, the financial burden of resolving a problem with AI may outweigh the cost of human labor. However, relying on humans in this context merely postpones the inevitable adoption of AI.
High-value work, which is the cornerstone of any successful business operation, deserves our attention. Every employee is often bombarded with requests from peers and external stakeholders. Amid these demands, it’s important to prioritize. Generative AI can help by taking on tedious tasks, freeing up individuals to focus on high-value projects that require creative and critical thinking.
Generative AI innovation accelerated
When reflecting on McKinsey’s previous reports, the advancement of natural language understanding technology surpasses all expectations. Previously, experts predicted that AI technology capable of human-like tasks would emerge in 2027. However, as of 2023, we are witnessing this transition happening right now. This leap signifies a four-year acceleration, which has tremendous implications for businesses worldwide.
What are the practical uses of Generative AI?
The question remains: How should businesses perceive and respond to this accelerated development? While some might dismiss it as mere hype, the practical use cases of AI, as outlined in various research papers, are too compelling to ignore.
In one instance, a Krista partner who sought ten potential use cases for AI from customers ended up identifying a hundred viable scenarios, each with a distinct dollar value. With the rapid advancement of technology, waiting and watching is no longer an option. If you don’t adopt AI now, your competitors will, leaving you trailing in their wake.
Generative AI is redefining businesses
As we move towards 2024, we can anticipate a significant shift in business budgets to accommodate the integration of generative AI. Businesses are already implementing or conducting pilots and realizing the immense value generative AI offers. Notably, implementing AI is not as complex as it may seem, provided you approach it the right way. Previous episodes on our generative AI integration methods underscore how swiftly you can actualize AI, govern your data, and automate the next steps of generative AI.
We are on the brink of an AI revolution. Businesses that wish to survive must embrace generative AI and start integrating it into their operations. The future is here.
Links and Resources
Speakers
Transcription
Scott King:
Welcome, everyone, to this episode of the Union Podcast. This episode will be a little different. We’re going to discuss the recent McKinsey report, titled The economic potential of generative AI: The next productivity frontier. It’s not about one model or one LLM but encompasses all of generative AI. We’ve reviewed the report and found it extremely interesting. We’ll walk through some of the findings. Chris, what do you want to emphasize to the audience before we dive into the details?
Chris Kraus:
Well, what stands out to me about this report is its depth. They’ve quantified different use cases across industries and provided valuable statistics on how these can be achieved. This report doesn’t seem like conjecture. You can see the research behind it, especially when you look at exhibit two and the bar charts comparing 2017 to today and the advancements in generative AI versus standard AI analytics. What I appreciate is that the report is well-written and not just fluff. They’ve shared significant statistics on how industries should adapt and evolve.
Scott King:
Yes, the McKinsey Global Institute does provide a comparison with the 2017 report. I’d love to be a fly on the wall during those meetings when they realized how generative AI has advanced and made their old report outdated. The impact is far greater now, right? We see the use cases. We’ve done a few different ChatGPT episodes, and when you understand how it could apply to your job and your team’s work, it’s eye-opening. They estimate an additional 2.6 to 4.6 trillion dollars per year, which is equivalent to the GDP of the UK.
That’s a considerable amount. I think the point you raised about the impact is crucial, Chris. They’ve quantified the use cases. In the report, they list these use cases in a chart on exhibit three, page 12. The top portions, they claim, are 75% of the impact. These include sales, marketing, software engineering, customer operations, and product R&D. What are your thoughts on some of those use cases?
Chris Kraus:
I believe the approach towards customer operations is fundamentally different. Traditionally, we focused on reducing costs incrementally in existing modes of business. However, the report suggests using generative AI, with its natural language understanding, to completely deflect the need for a human interaction, thus saving the entire cost of the call. This is a departure from the typical function of FAQs and chatbots, which often serve merely as placeholders before you speak to a person. If generative AI can accurately understand your question and guide you, that’s a game-changer. It could increase customer satisfaction significantly, as we accomplish more with the same resources.
Scott King:
I agree. It would be beneficial to eliminate the call entirely, especially sales-related calls. For instance, I recently had an experience where I had a very specific question regarding API rate limits for a vendor we use. Despite being forced to speak with a salesperson, they didn’t understand what an API was or how to assist me. It was a situation that could’ve been handled through an automated response. Simple keyword and entity understanding could’ve resolved my query without involving a salesperson.
Chris Kraus:
Absolutely. We’ve worked with both large and small companies, and they usually have specialized individuals to address specific areas. However, as computers have become faster and more efficient, an AI model can master several different functional areas. An individual might not understand all aspects of a product, but a bot, using natural language understanding and generation, can be trained across all disciplines within a company. This could provide faster and more accurate responses. Humans can’t memorize everything. We’re either generalists, knowing a little about a lot, or specialists in one area. With software, we can be both.
Scott King:
Software indeed offers quicker memory recall. We remember everything but often forget where we’ve stored it. So the question of customer service and operations is one of the more straightforward use cases. We understand the frustrations with chatbots because people often ask us the difference between Krista and a chatbot. The difference is understanding. So, while marketing and sales are high use cases and generative AI is efficient at creating content, our focus is on enterprise IT operations. The report mentions software engineering as a significant use case. Chris, could you walk us through some of those use cases and how they would work?
How can generative AI be used for software engineering?
Chris Kraus:
There’s often apprehension around adopting new technology, but when it comes to software engineers using an AI copilot for coding, it seems well received. We’ve had paired programming for years, and now the idea is to replace the second human programmer with an AI copilot. We’ve also had autocomplete for classes and functions for some time now. Given that software engineers tend to enjoy trying out new technologies, they’re not as concerned about AI replacing their jobs. They understand that you still need to instruct the AI on what to do. This is why the diagram on page 20 highlights all these different areas. The tech people who adopt and use new technology are not afraid of it, which is why we see traction in these areas. AI coding copilots make sense, as they can help spot possible missed cases during coding and assist with testing, a task which can often be rushed due to lack of time. The report even goes so far as to discuss maintenance, which is relevant to our use case with Zimperium. When dealing with large log files, we need to be able to consolidate errors, summarize them quickly, and draw conclusions. So, the fact that this technology is being embraced by tech-savvy individuals is not surprising.
Scott King:
Exactly. As a software engineer, I would lean on the “hallucination case study” examples to reassure myself that while AI can assist me, it won’t take over my understanding of how the code works. And as you said, there have been code generation tools for a long time. Software is omnipresent, so it’s only going to improve. The report also touches on Research and Development, which might be slightly out of our depth, but let’s discuss that. What were their insights on R&D?
Chris Kraus:
They highlighted the use of complex algorithms and how computing is getting faster, specifically with regard to GPU performance and computer threading models. This acceleration is particularly useful in fields like pharmaceutical research. They noted that while these complex algorithms have been around for a while, they used to take a long time to process. Now, with hardware advancements complementing AI, these computations can be performed much more quickly, even in real time. The report did a great job of outlining different use cases, from the improvement of CPUs and GPUs to AI assistance in various areas such as content creation, sales and marketing, and more. All these points seemed tangible and reasonable to me. It’s not a vision for the distant future, but something we should be considering right now, in the next 10 days even.
Scott King:
The report outlines the top 75% of areas where AI has the most impact. The bottom 25% of this list, where AI has a lesser impact in terms of functional spend, includes procurement, risk and compliance, legal, finance, manufacturing, manufacturing strategy, and pricing. I was surprised to see risk and compliance on this list.
Chris Kraus:
Yes, it’s unexpected.
Scott King:
Maybe the report isn’t considering risk aversion as a potential gain from AI in finance, given it’s a complicated area dealing with audits, suppliers, and potentially thousands of third-party suppliers for large companies. What are your thoughts on risk and compliance?
How can generative AI be used in risk and compliance?
Chris Kraus:
We’d probably disagree with the report’s view. In fact, Sam’s done a podcast with you on it (Challenges with Third-Party Risk Assessments). The report seems to focus on training specific language models on a body of information to answer questions in areas like finance. For instance, when someone asks, “What were my sales this quarter compared to last quarter?” That’s not something that you can train a model to answer based on historical data. Instead, you’d want to use automation to gather real-time data from backend systems. The training of models is beneficial for understanding the specifics of language in different contexts, like legal versus procurement. However, the report seems to view these areas as lower impact because it assumes a traditional approach of training models based on company-specific data. We discussed in our last podcast how a different approach could yield better results. If we look at these areas through a different lens, where the large language model understands the discussion but other means provide the necessary data, then these areas could have more impact.
Scott King:
I’m referring to exhibit four on page 25, which covers risk, legal, and supply chain. Supply chain is closely tied to risk and legal aspects, considering third-party risks. Looking at the industries with the most complexity like banking, energy, insurance, real estate, telecommunications, they have complicated contracts and large customer bases. This is why I think risk and compliance should be higher on the list. We use automation to find data, which then informs the AI to ask the right questions. It’s a different and more cost-effective approach compared to training a model to answer questions. This is what we do at Krista. It’s surprising to see these areas ranked low. Then, the report moves on to the types of work that generative AI is estimated to have the most impact on. What are your thoughts, Chris?
What types of work will be impacted by generative AI?
Chris Kraus:
They’re distinguishing between back office versus front office, customer-facing aspects such as a call center handling complaints and orders, and back office operations within a bank. The interesting thing is that the areas you’re discussing are actually customer-facing. It’s customer service, but for a backend process within the bank. I think that certain tasks like having the AI ensure all necessary documents are in place before it goes to the loan officer for approval can be automated. The AI can scan the documents, identify what each one is, and make a suggestion for a human to approve. This kind of assistance from AI would help professionals eliminate mundane tasks and focus more on the essentials. If the AI is trained on your specific model, it won’t deviate from these rules and policies.
Scott King:
There’s also the consideration of how different situations in various parts of the world may affect the cost-benefit analysis. Sometimes, solving the problem can be more expensive than the labor to do it, especially in lower-wage countries.
Chris Kraus:
That’s true. Cost center agents tend to be paid less, which presents a good cost model compared to a bank officer in a local branch. However, if we train generative AI on the tasks a cost center agent needs to know, and they can automate most of these tasks, there’s a lot of value in that. More educated people can then focus on using their brainpower to do educated tasks rather than ensuring all the forms are filled out.
Scott King:
It’s about prioritizing high-value work. Everyone has a list of tasks, and if we can automate the lower-priority tasks, it adds enormous value. That’s more the focus of the report, which leans towards high-knowledge workers.
Chris Kraus:
Absolutely.
Scott King:
This brings us to the last exhibit that I want to talk about: exhibit six. According to this, due to the advancements in generative AI, the estimates from McKinsey’s 2017 report have been completely revised. They’re now saying that the median human capability for this technology, which was initially expected in 2027, is available today. How do you think people will respond to that, and what should they do?
Chris Kraus:
Some may dismiss it as hype, but this paper does an excellent job of illustrating its practicality with real use cases. People are intrigued by chatbot technology but also wary of potential issues. We’re getting questions on how to operationalize this technology. One of our partners queried customers for use cases, expecting about ten, but they ended up with a hundred valuable use cases. So, the need to start adopting this technology is now, not later, as the date has moved forward from 2027 to 2023.
Scott King:
Certainly, budgets for 2024 will likely be shifted towards this, especially since the technology is valuable, achievable, and simpler than anticipated. We’ve previously discussed our methods for integrating generative AI and how automation is crucial for its next steps, and we’ll link back to that. Any final thoughts, Chris?
Chris Kraus:
No, all good here.
Scott King:
I appreciate your input, Chris. Thanks, everyone. Until next time.
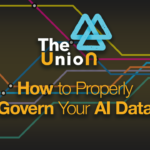
Read our comprehensive LLM testing guide
See how several LLMs fared in our test and steps to run your own tests for each of your use cases.
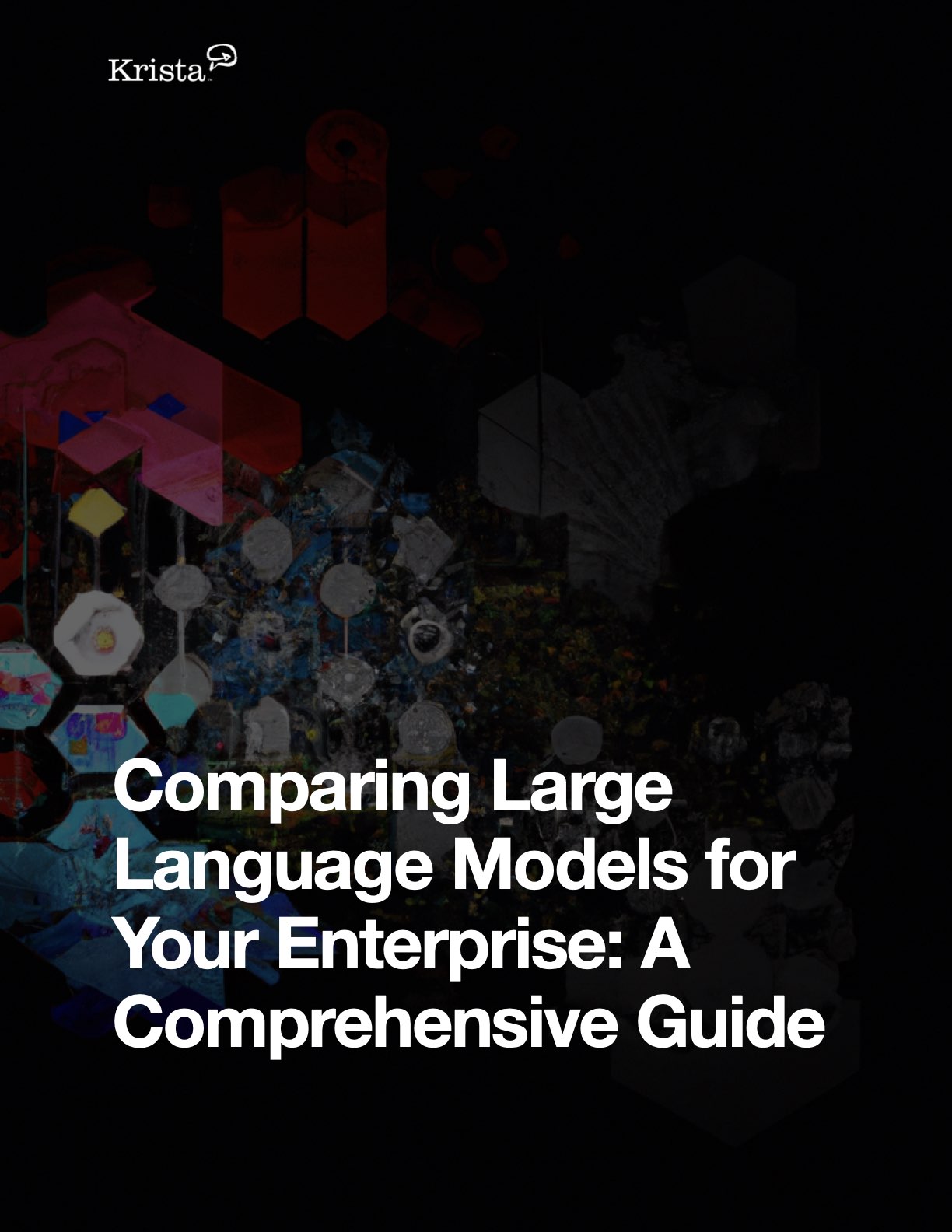